Knowledge 4 All Foundation Completes NLP Projects with Lacuna Fund, Transitions Efforts to Deep Learning Indaba Charity
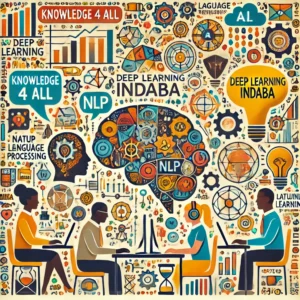
The Knowledge 4 All Foundation is pleased to announce the successful completion of its Natural Language Processing (NLP) projects under the Lacuna Fund initiative. These projects aimed to develop open and accessible datasets for machine learning applications, focusing on low-resource languages and cultures in Africa and Latin America.
The portfolio includes impactful initiatives such as NaijaVoice, which focuses on creating datasets for Nigerian languages, Masakhane Natural Language Understanding, which advances NLU capabilities for African languages, and Masakhane Domain Adaptation in Machine Translation, targeting improved domain-specific machine translation systems. The Foundation’s efforts have significantly contributed to assisting African researchers and research institutions in creating inclusive datasets that address critical needs in these regions.
As part of a strategic transition, the Foundation has entrusted the continuation and expansion of these initiatives to the Deep Learning Indaba charity. The Deep Learning Indaba, dedicated to strengthening machine learning and artificial intelligence across Africa, is well-positioned to build upon the groundwork laid by Knowledge 4 All. The Foundation extends its gratitude to the Deep Learning Indaba charity for taking over these projects and is confident that their expertise will further the mission of fostering inclusive and representative AI development in the future.