Description
This dataset is part of a 3-4 month Fellowship Program within the AI4D – African Language Program, which was conceptualized as part of a roadmap to work towards better integration of African languages on digital platforms, in aid of lowering the barrier of entry for African participation in the digital economy.
This particular dataset is being developed through a process covering a variety of languages and NLP tasks, in particular Machine Translation of Fongbe.
Language profile: Fongbe
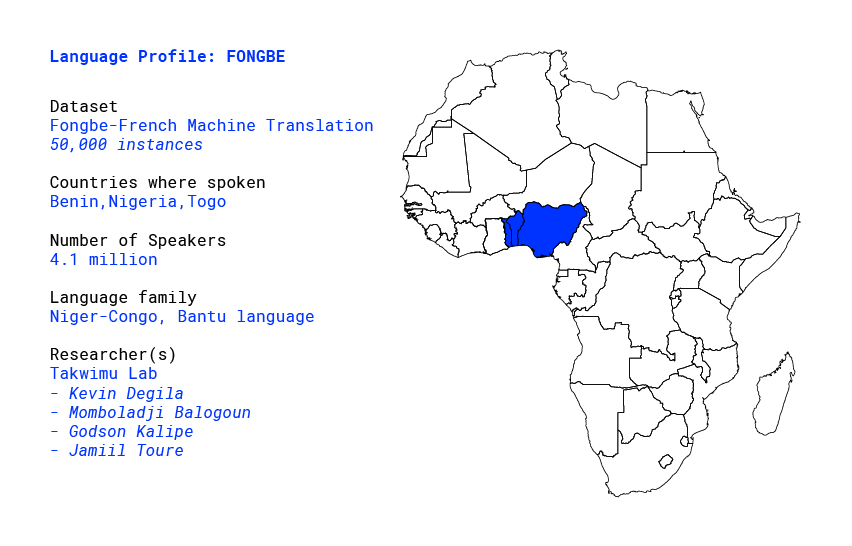
Overview
Fon or fɔ̀ngbè is a low resource language, part of the Eastern Gbe language cluster and
belongs to the Volta–Niger branch of the Niger–Congo languages. Fongbe is spoken in Nigeria, Togo and mainly in Benin by approximately 4.1 million speakers. Like the other Gbe languages, Fongbe is an analytic language with an SVO basic word order. It’s also a tonal language and contains diacritics which makes it difficult to study. [1]
The standardized Fongbe language is part of the Fongbe cluster of languages inside the Eastern Gbe languages. In that cluster, there are other languages like Goun, Maxi, Weme, Kpase which share a lot of vocabulary with the Fongbe language. Standard Fongbe is the primary target of language planning efforts in Benin, although separate efforts exist for Goun, Gen, and other languages of the country. To date, there are about 53 different dialects of the Fon language spoken throughout Benin.
Pertinence
Fongbe holds a special place in the socio economic scene in Benin. It’s the most used language in markets, health care centers, social gatherings, churches, banks, etc.. Most of the ads and some programs on National Television are in Fongbe. French used to be the only language of education in Benin, but in the second decade of the twenty first century, the government is experimenting with teaching some subjects in Benin schools in the country’s local languages, among them Fongbe.
Example of Fongbe Text:
Fongbe : Mǐ kplɔ́n bo xlɛ́ ɖɔ mǐ yí wǎn nú mɛ ɖevo lɛ
English : We have learned to show love to others [3]
Existing Work
Some previous work has been done on the language. There are doctorate thesis, books, French to Fongbe and Fongbe to French dictionaries, blogs and others. Those are sources for written fongbe language.
Researcher Profile: Kevin Degila
Kevin is a Machine Learning Research Engineer at Konta, an AI startup based in Casablanca. he holds an engineering degree in Big Data and AI and it’s currently enrolled in a PhD program focused on business document understanding at Chouaib Doukkali University. In his day to day activities, Kevin train, deploy and monitor in production machine learning models. With his friends, they lead TakwimuLab, an organisation working on training the next young, french speaking, west africans talents in AI and solving real-life problems with their AI skills. In his spare time, Kevin also create programming and AI educational content on Youtube and play video games.
Researcher Profile: Momboladji Balogoun
Momboladji BALOGOUN is the Data Analyst of Gozem, a company providing ride-hailing and other services in West and Central Africa. He is a former Data Scientist at Rintio, an IT startup based in Benin, that uses data and AI to create business solutions for other enterprises. Momboladji holds a M.Sc. degree in Applied Statistics from ICMPA UNESCO Chair, Cotonou, and migrated to the Data Science field after having attended a regional Big Data Bootcamp in his country Benin. He aims to pursue a Ph.D. program on low resources languages speech to speech translation. Bola created Takwimu LAB in August 2019, and he leads it currently with 3 other friends in order to promote Data Science in their countries, but also the creation and the use of AI to solve real-life problems in their communities. His hobbies are: Reading, Documentaries, and Tourism.
Researcher Profile: Godson Kalipe
Godson started in the IT field with software engineering with a specialization on mobile applications. After his bachelor in 2015, he worked for a year as web and mobile application developer before joining a master in India in Big Data Analytics. His master thesis consisted comparative analysis of international news impact on economic indicators of African countries using news Data, Google Cloud storage and visualization assets. After his Master,
in 2019, he gained a first experience as Data Engineer creating data ingestion pipelines for real time sensor data at Activa Inc, India. He parallely has been working with Takwimu Lab on various projects with the aim of bringing AI powered solutions to common african problems and make the field more popular in the west African francophone industry.
Researcher Profile: Jamiil Toure
Jamiil is a design engineer in electrical engineering from Ecole Polytechnique d’Abomey-Calavi (EPAC), Benin in 2015 and a master graduate in mathematical sciences from African School of Mathematical Sciences (AIMS) Senegal in 2018. Passionate of languages and Natural Language Processing (NLP), he contributes to the Masakhane project by working on the creation of a dataset for the language Dendi.
Meanwhile, he complements his education on NLP concepts via online courses, events, conferences for a future research career in NLP. With his friends at Takwimu Lab they work at creating active learning and working environments to foster the applications and usages of AI to tackle real-life problems. Currently, Jamiil is a consultant in Big Data at Cepei – a think tank based in Bogota that promotes dialogue, debate, knowledge and multi-stakeholder participation in global agendas and sustainable development.
Partners
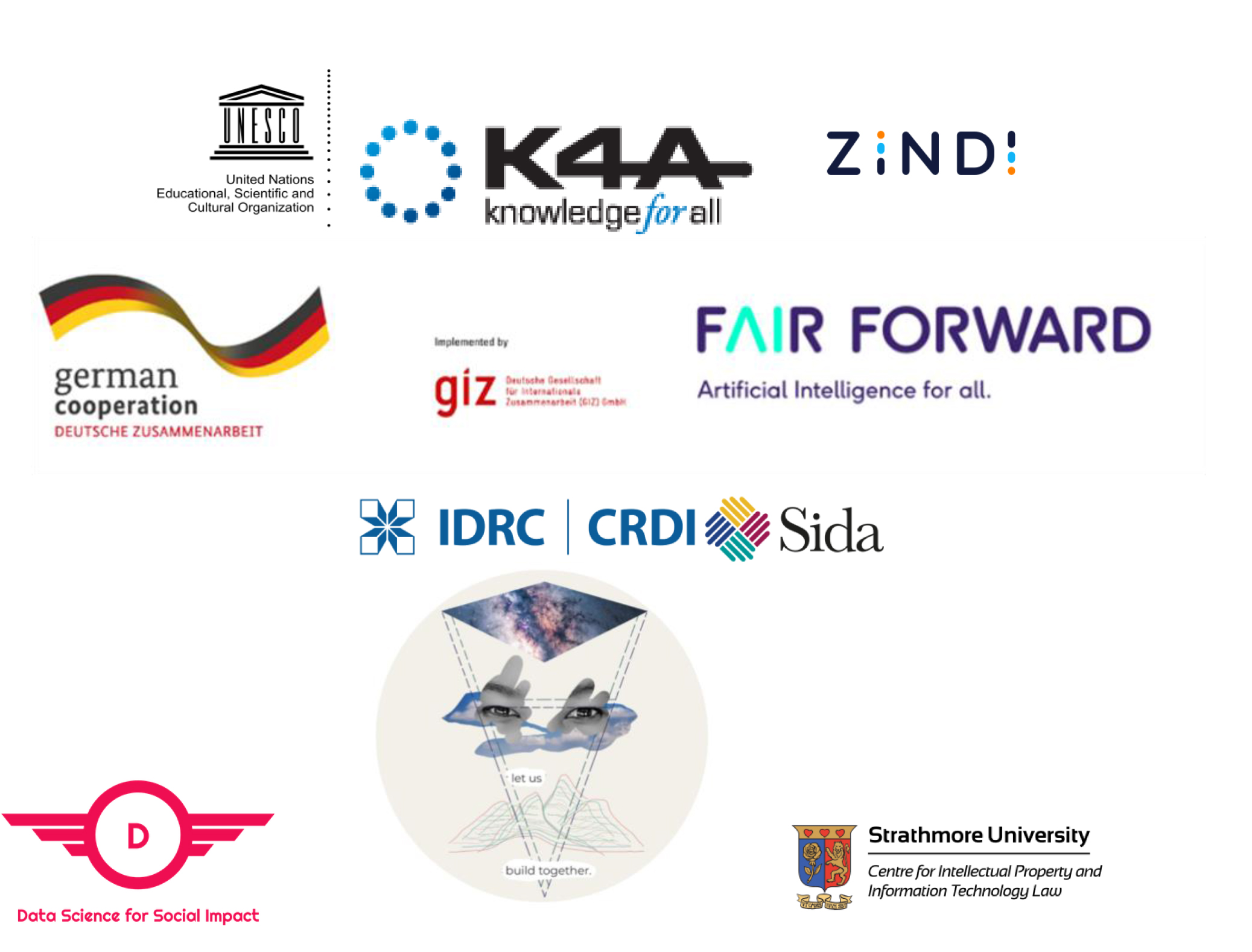
Disclaimer
The designations employed and the presentation of material on these map do not imply the expression of any opinion whatsoever on the part of the Secretariat of the United Nations concerning the legal status of any country, territory, city or any area or of its authorities, or concerning the delimitation of its frontiers or boundaries. Final boundary between the Republic of Sudan and the Republic of South Sudan has not yet been determined. Final status of the Abyei area is not yet determined.