What is the overall scope of the project?
The project will deliver three main components from research in natural language processing, dataset creation, and policy creation:
- Fellowship for African AI researchers focused on African languages, based on previously funded work on language datasets. This work contributes to a roadmap for better integration of African languages on digital platforms in aid of lowering the barrier for African participation in the digital economy,
- Improvement of the representation of AI research carried out on African languages by creating resources for a variety of NLP tasks and in a variety of African languages that will enable good, data-driven results in AI research,
- Attract an African community of native speakers as contributors of language resources and language technology tools to adopt and support Masakhane NLP, a platform for sharing, maintaining and making use of language resources and tools; establishing widely agreed benchmarks for NLP tasks and stimulating competition between methods and systems,
- Be used as a model case to inform African evidence-based policymaking concerning Artificial Intelligence and will be included in UNESCO’s AI Decision maker’s Essential to inform policymakers.
This project is lead by 29 researchers, covers 9 African languages, spoken across 22 countries, reaching 300 million speakers
What are the results at the moment?
Result 1: African Language Datasets
Below, we detail the languages of datasets created. Delivering the project Cracking the Language Barrier for a Multilingual Africa[1] includes a Fellowship for Low Resource African Languages in order to develop datasets and strengthen capacities and innovation by building specific datasets[2]:
- Ewe language[3] and Fongbe language[4] parallel text dataset for NM Translation
- Yoruba language[5] Machine Translation dataset
- Chichewa language[6] document classification datasets
- Wolof language[7] (link) text-to-speech dataset for
- Kiswahili language[8] (link) document classification datasets
- Tunisian Arabizi language[9] sentiment analysis dataset
- Swahili: News Classification Dataset[10]
- Twi language[11]
- Luganda language[12]
Result 2: African Language Dataset Challenges
These AI4D datasets are further made into competitions of five NLP challenges hosted on Zindi as part of this AI4D’s ongoing African language NLP project, which is a continuation of the African language dataset challenges we hosted in 2020[13]. The competition engagement in the challenges shows a total of 153,926 unique page views across 111 countries for the implementation of the challenges: Tunizi Arabizi, Yoruba, Ewe & Fongbe, Chichewa. Wolof went live on 12 Feb 2020 so statistics are not available yet. The current statistics of each challenge are the following:
- AI4D iCompass Social Media Sentiment Analysis for Tunisian Arabizi objective[17] (20 November 2020—29 March 2021): 539 Data scientists enrolled, 213 Data scientists on the leaderboard, 3 630 Submissions, Accuracy score: 0.94
- AI4D Malawi News Classification Challenge challenge[18] (22 January—10 May): 218 Data scientists enrolled, 69 Data scientists on the leaderboard, 686 Submissions, Accuracy score: 0.64
- AI4D Takwimu Lab – Machine Translation Challenge challenge[19] (18 December 2020—26 April 2021): 134 Data scientists enrolled, 11 Data scientists on the leaderboard, 142 Submissions, BLEU score: 0.35
- AI4D Yorùbá Machine Translation Challenge challenge[20] (4 December 2020—12 April 2021): 314 Data scientists enrolled, 33 Data scientists on the leaderboard, 285 Submissions, BLEU score: 0.43
- AI4D Baamtu Datamation – Automatic Speech Recognition in WOLOF challenge[21] (12 February—24 May)
Result 3: Speech-to-text Platform for African Languages
The objective of this third part of the project is to build a Wolof text-to-speech system, to be extended into a general platform for all African languages as part of the Masakhane platform. The project will exploit a dataset of 40000 Wolof phrases uttered by two actors. This open-source dataset is a deliverable of a previous project. The project will be conducted following four phases:
- Evaluation of the quality of the dataset
- Implementation of a machine learning model mapping Wolof texts into their corresponding utterances
- Quantitative and qualitative evaluation of the implemented model’s performances
- Development of an API exposing implemented text to speech model
What are we specifically trying to achieve?
Advances in speech and language technologies now enable tools such as voice search, text-to-speech, speech recognition and machine translation. These are however only available for high resource languages like English or Chinese.
Without foundational digital resources for African languages, which are considered low-resource in the digital context, these advanced tools remain out of reach. This work details the AI4D – African Language Program, a 3-part project that will:
- Incentivise the crowd-sourcing, collection and curation of language datasets through an online quantitative and qualitative challenge
- Support research fellows for a period of 3-4 months to create datasets annotated for NLP tasks
- Host competitive Machine Learning challenges on the basis of these datasets.
How do Language Technologies relate to UNESCO?
Languages, with their complex implications for identity, cultural diversity, spirituality, communication, social integration, education and development, are of crucial importance for people, prosperity and the planet.
People not only embed in languages their history, traditions, memory, traditional knowledge, unique modes of thinking, meaning and expression, but more importantly they also construct their future through them.
In this context, Language Technologies (LT), greatly contribute to the promotion of linguistic diversity and multilingualism. These technologies are moving outside research laboratories into numerous applications in many different areas. UNESCO’s International Conference Language Technologies for All (LT4All): Enabling Linguistic Diversity and Multilingualism Worldwide, organized in December 2019, underlined spelling/grammar checkers up to speech and speaker recognition, machine translation for text and audio, speech synthesis, and spoken dialogue among others as important areas for enabling linguistic diversity and multilingualism.
In addition, the Los Pinos Declaration on the Decade of Indigenous Languages (2022-2032) calls for the design and access to sustainable, accessible, workable and affordable language technologies and places indigenous peoples at the centre of its recommendations under the slogan “Nothing for us without us.”
What is the African Languages Programme?
The AI4D – African Language Program was conceptualized as part of a roadmap to work towards better integration of African languages on digital platforms, in aid of lowering the barrier of entry for African participation in the digital economy. It was organised in 3 key phases.
Phase 1: Language Dataset Challenges
The AI4D Language Dataset Challenges were framed to focus on data collection, in response to the challenges of low availability of input data for African languages and the poor discoverability of resources that do exist, thus hindering the ability of researchers to do machine translation [14], and other NLP tasks.
We put together a panel of judges and performed both qualitative and quantitative evaluations, based on datasheets [2] submitted with each datasets and the datasets themselves, to identify outstanding submissions. The two rounds of this challenge yielded 52 dataset submissions with 13 winning prizes.
Some of the key observations identified from this phase of the project include [3]:
- Teams composed of individuals from relevant multi-disciplinary backgrounds, including computer scientists, professional translators and linguists, were able to create and annotate datasets that captured fundamental lexical and semantic nuances of languages.
- The challenge framing allowed for anyone to participate. While useful as an exercise in evaluating the interest in such a challenge, high quality submissions came from teams who had been exposed to NLP research work.
- Since the challenge was evaluated monthly, we often received disparate submissions from the same teams. Instead, one large dataset built over a couple of months would have been the ideal outcome.
These observations motivated the design of a subsequent phase of the project, the Fellowship.
Phase 2: Language Dataset Fellowships
From the top teams that participated in the challenges, we invited nine to take part in a subsequent phase of the program, a 3-4 month Fellowship Program. This provided research grants for teams to invest in resources, gave enough time for collaborative consultations to determine the sizes of expected datasets, the downstream NLP tasks they would be annotated for as well as mentorship and advisory requirements.
The datasets developed through this process cover a variety of languages and NLP tasks; Machine Translation datasets (Ewe, Fonge, Yoruba, Luganda, Twi and the 11 official languages of South Africa), a Text-to-Speech dataset (Wolof), a Sentiment Analysis dataset (Tunisian Arabizi), a Keyword Spotting dataset (Luganda) and Document Classification datasets (Chichewa and Kiswahili).
The Fellowship also presented a platform to tackle some opportunities identified to support future work in African, and low resource, language dataset creation, including research and analysis of the legal implications of obtaining textual, visual and audio data from a variety of online sources, and the development of copyright, intellectual property and data protection guidelines for NLP researchers.
These guidelines will be published in addition to research papers from the individual fellows on their particular dataset development work.
Phase 3: Machine Learning Competitions
This final phase, which is still in progress, will involve design and split of the datasets into train, development and test sets; the preparation of datasheets to document the motivation, composition, collection process and recommended uses of the datasets and hosting ML competitions on Zindi, an African data science competition platform, so as to engage the wider NLP community.
What is our research ambition?
The objective of this work is to create good quality African language datasets for a variety of Natural Language Processing and Speech Processing tasks. This work is in support of Masakhane, a research effort for NLP for African languages. Masakhane as a resource is open source, continent-wide, distributed and online. It is a community of researchers working on a wide variety of African languages, some of whom are funded to build datasets via this AI4D fellowship.
Some of the challenges for the development of NLP for African languages identified by researchers in Africa include (Martinus and Abbott 2019):
- Low availability of resources (input data) for African languages that hinders the ability for researchers to do machine translation.
- Discoverability: The resources for African languages that do exist are hard to find. Often these resources are not available under open access licenses thus reducing the ability of research institutions to work together and share knowledge on language datasets to strengthen innovation.
- Reproducibility: The data and code of existing research are rarely shared, which means researchers cannot reproduce the results properly.
- Lack of benchmarks: Due to the low discoverability and the lack of research in the field, there are no publicly available benchmarks or leader boards to compare new machine translation techniques to old ones.
What kind of impact do we want to achieve?
The project aims at addressing some of the challenges identified above through:
- Development of datasets of African languages that maybe used in some countries only or have transboundary usage that can be used for strengthening access to information and spur innovation based on NLP technologies
- Enhancement of capacities among young researchers for the development of open languages datasets and language tech applications through development of guidelines and training through open educational resources in collaboration with national institutions
- Development of a multi stakeholder network for strengthening research on language technology based on AI techniques for African languages
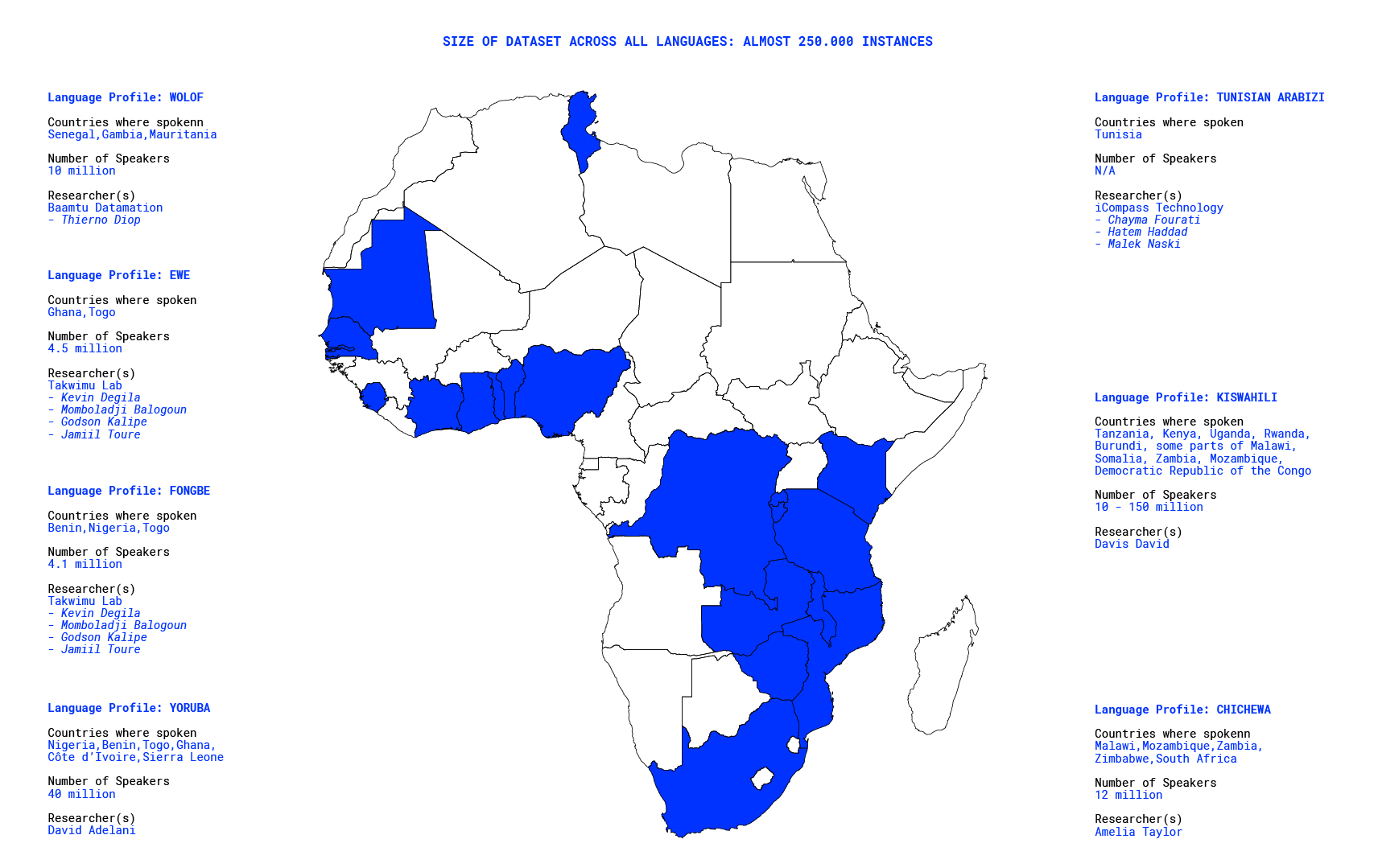
How do we plan on implementing this project?
Supported by AI4D-Africa and the University of Pretoria’s Data Science for Social Impact Research Group and Knowledge 4 All Foundation, the first month will involve consultations alongside the teams to determine a number of factors:
- Dataset
- Language
- Downstream task scoping
- Expected sizes of datasets
- Preparation and documentation process
- Deliverables
- Monthly targets and check-ins for guidance
- Workshop/Conference paper for publication documenting the process
The teams will then have 3 months to further flesh out their datasets, after which, they will be expected to prepare write-ups of their research for publication. Once the dataset creation phase is complete, the datasets will be used in ML challenges hosted in Zindi and evaluated on the downstream task that each dataset has been prepared for. UNESCO funding will be used to promote the work of Fellows 1-5, and GIZ funding will be used to promote Fellows 6-8.
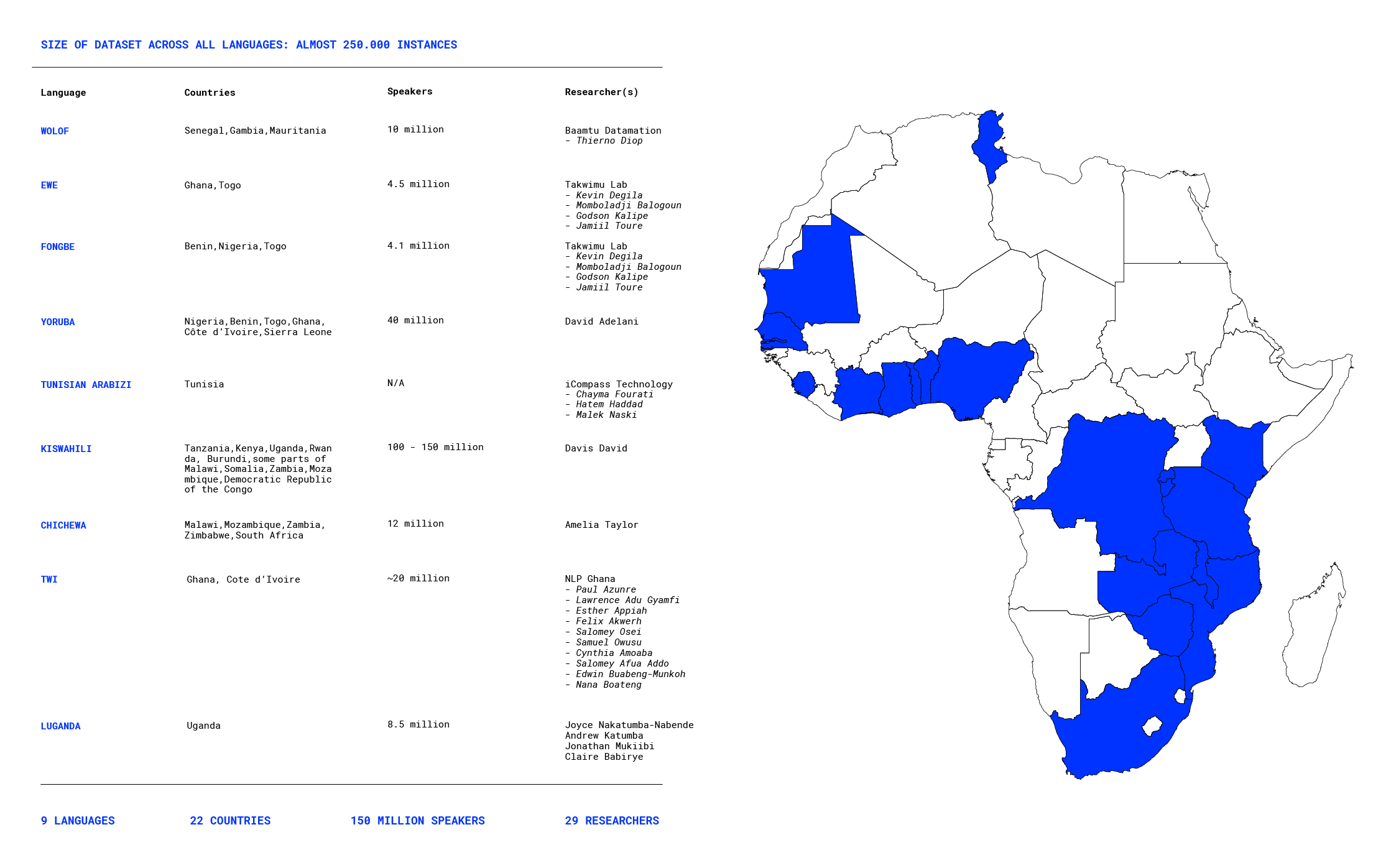
Who are the Fellows?
- Fellow 1: Amelia Taylor – Chichewa language
- Fellow 2: Takwimu Lab – Fongbe and Ewe language
- Fellow 3: David Adelani – Yoruba language
- Fellow 4: Baamtu Datamation –Wolof language
- Fellow 5: iCompass – Tunisian Arabizi language
- Fellow 6: Davis David – Kiswahili language
- Fellow 7: Ari Ramkilowan and Masabata Mokgesi-Selinga – the 11 national South African languages
- Reseach Fellow 8: Makerere University – Luganda language
- Fellow 9: GhanaNLP – Twi language
What are the partners involved?
This work has been sponsored through a partnership between several organisations, listed below in non-alphabetical rder;
- Knowledge 4 All Foundation
- AI4D Africa Initiative
- Zindi platform
- Data Science for Social Impact Research Group, University of Pretoria (DFSI)
- Centre for Intellectual Property and Information Technology (CIPIT), Strathmore University
- United Nations Educational, Scientific and Cultural Organization (UNESCO)
- GIZ – Deutsche Gesellschaft für Internationale Zusammenarbeit (GIZ) GmbH
- IDRC – International Development Research Centre
- UNESCO Chair in Artificial Intelligence at University College London
References
[1] Cracking the Language Barrier for a Multilingual Africa https://www.k4all.org/project/language-dataset-fellowship/
[2] African Natural Language Processing https://zenodo.org/communities/africanlp/?page=1&size=20
[3] Ewe language https://www.k4all.org/project/ewe-database/
[4] Fongbe language https://www.k4all.org/project/database-fongbe/
[5] Yoruba language https://www.k4all.org/project/database-yoruba/
[6] Chichewa language https://www.k4all.org/project/database-chichewa/
[7] Wolof language https://www.k4all.org/project/database-wolof/
[8] Kiswahili language https://www.k4all.org/project/database-kiswahili/
[9] Tunisian Arabizi language https://www.k4all.org/project/database-tunisian-arabizi/
[10] Swahili language https://www.k4all.org/project/database-kiswahili/
[11] Twi language https://www.k4all.org/project/twi-language/
[12] Luganda language https://www.k4all.org/project/luganda-language/
[16] Zindi and AI4D build language datasets for African NLP https://zindi.medium.com/zindi-and-ai4d-build-language-datasets-for-african-nlp-34a4d0ea129
[17] AI4D iCompass Social Media Sentiment Analysis for Tunisian Arabizi https://zindi.africa/competitions/ai4d-icompass-social-media-sentiment-analysis-for-tunisian-arabizi
[18] AI4D Malawi News Classification Challenge https://zindi.africa/competitions/ai4d-malawi-news-classification-challenge
[19] AI4D Takwimu Lab – Machine Translation Challenge https://zindi.africa/competitions/ai4d-takwimu-lab-machine-translation-challenge
[20] AI4D Yorùbá Machine Translation Challenge https://zindi.africa/competitions/ai4d-yoruba-machine-translation-challenge
[21] AI4D Baamtu Datamation – Automatic Speech Recognition in WOLOF https://zindi.africa/competitions/ai4d-baamtu-datamation-automatic-speech-recognition-in-wolof
[1] Martinus, J. Webster, J. Moonsamy, M. S. Jnr, R. Moosa, and R. Fairon. Neural machine translation for south africa’s official languages. arXiv preprint arXiv:2005.06609, 2020.
[2] Gebru, J. Morgenstern, B. Vecchione, J. W. Vaughan, H. Wallach, H. Daumé III, and K. Crawford. Datasheets for datasets. arXiv preprint arXiv:1803.09010, 2018.
[3] Siminyu, S. Freshia, J. Abbott, and V. Marivate. Ai4d–african language dataset challenge. arXiv preprint arXiv:2007.11865, 2020.
Partners
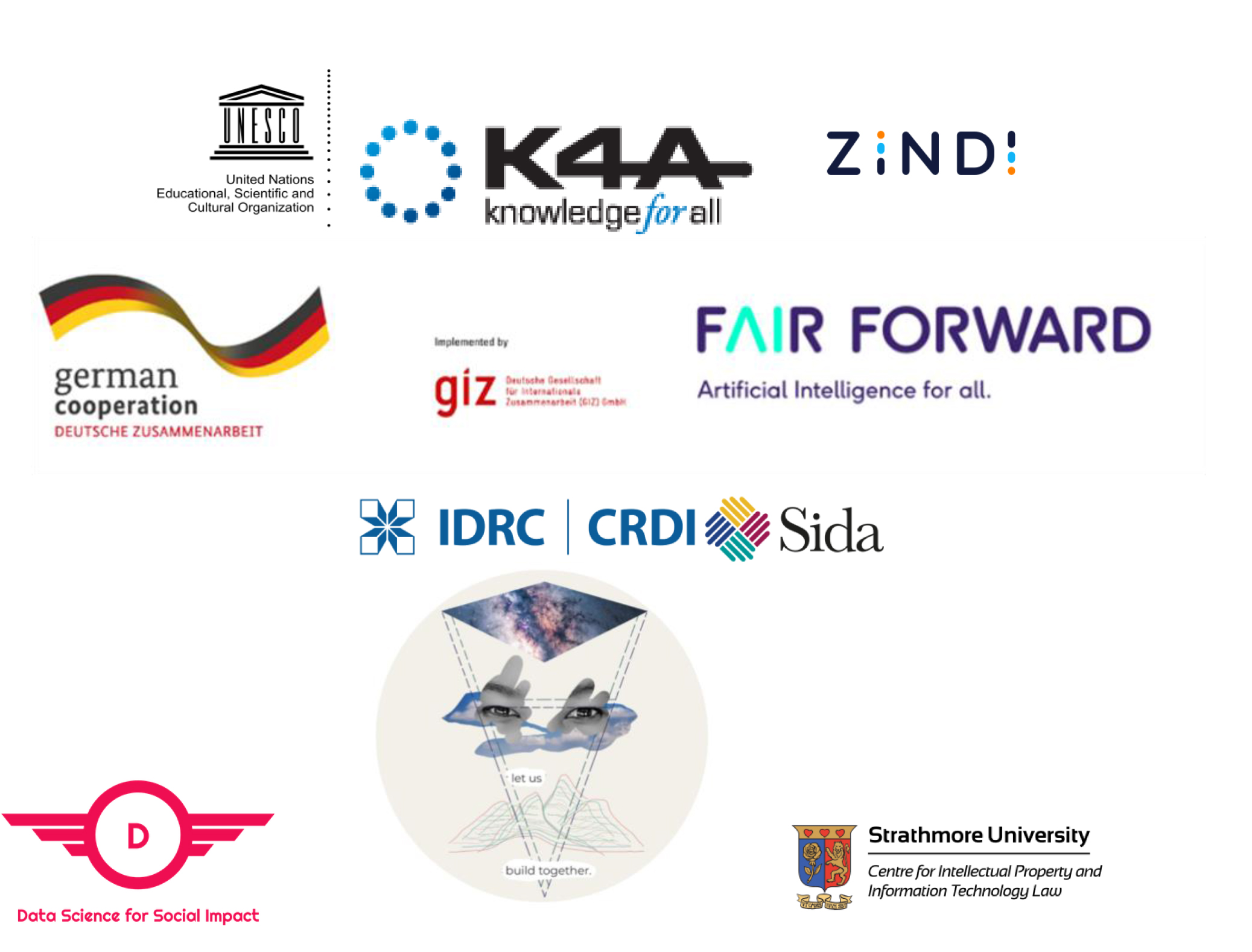
Disclaimer
The designations employed and the presentation of material on these map do not imply the expression of any opinion whatsoever on the part of the Secretariat of the United Nations concerning the legal status of any country, territory, city or any area or of its authorities, or concerning the delimitation of its frontiers or boundaries. Final boundary between the Republic of Sudan and the Republic of South Sudan has not yet been determined. Final status of the Abyei area is not yet determined.
Language profile: Ewe
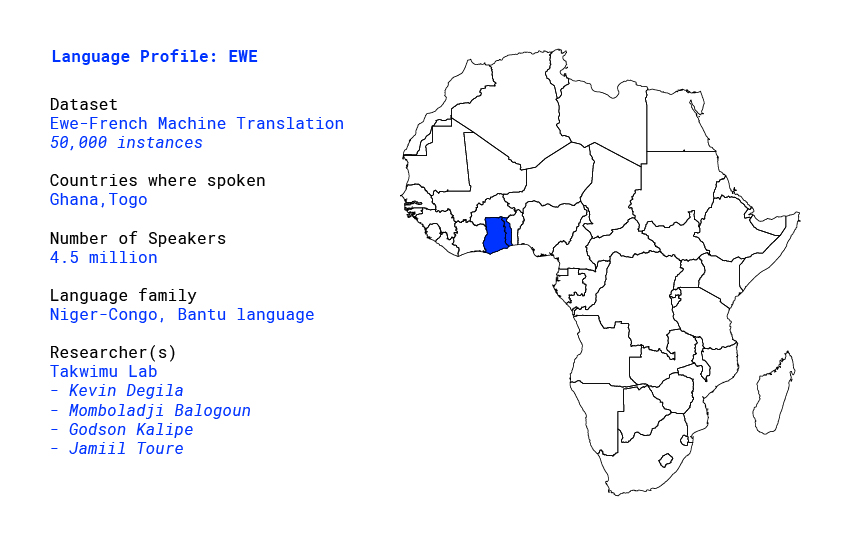
Overview
Ewe (Èʋe or Èʋegbe [èβeɡ͡be]) is a Niger–Congo language spoken in Togo and southeastern Ghana by approximately 4.5 million people as a first language and a million or so more as a second language.[1] Ewe is part of a cluster of related languages commonly called Gbe; the other major Gbe language is Fon of Benin. Like many African languages, Ewe is tonal.
Pertinence
In Togo and Ghana where ewe is heavily spoken, it is the main communication medium in major economic hubs especially in Togo where it is the most spoken language in the capital city Lome and also one of the two national languages of the country. In Ghana, ewe is part of the 11 government-sponsored languages apart from the official language english.[2] In 2020, the majority of children growing up in major cities in the Togo still picks up as their first language, a dialect of ewe depending on the region of the country they are from. The majority of the speakers at this day can speak ewe but not write it with the appropriate alphabet and orthography. The written communications in ewe usually happen using the english/french alphabet to write the sounds made by the words. Some schools in the capital offer ewe courses at secondary school level but those are generally optional and focus only on basics.
Nevertheless, in Togo, while the communication in schools and formally registered companies takes place in french, ewe remains the most used language in critical settings such as :
- Market places
- Medical centers
- In apprenticeship for a major array of occupations such as hairdressing, tailoring, engine repairing, carpenting, agriculture among other manual jobs that make up 90% of the jobs and 30% of the GDP of the country. [3]
- at police stations
- in banking or telecommunications agencies
- in shops and restaurants
Existing Work
Apart from sparse efforts of actors in the academic and literary fields and from some associations, there has not been any federated effort from the togolese government. Wycliffe-togo [4] is however one of the most prominent associations in the country organizing events and doing work to promote local languages. There exist a few ewe-english/french dictionaries online but the most popular ones remain the glosbe dictionary on the web [5], the Kasahorow Evegbe English Dictionary [6] and the mobile Ewe Dictionary [7] on the Android play store. In the academic world, a lot of work has been done especially regarding the tone, the syntax but also on other aspects such as the anthropological, lexicographical and phonological domains by both foreign and local (ghanain) researchers. [1]
Example of sentence in Ewe
Ewe : Ne ati aɖe le nya dim ɣesiaɣi le fíá wo ŋuti la, mumu ye le dzrom.
English : A tree which provokes axes wishes to be cut down.
Researcher Profile: Kevin Degila
Kevin is a Machine Learning Research Engineer at Konta, an AI startup based in Casablanca. he holds an engineering degree in Big Data and AI and it’s currently enrolled in a PhD program focused on business document understanding at Chouaib Doukkali University. In his day to day activities, Kevin train, deploy and monitor in production machine learning models. With his friends, they lead TakwimuLab, an organisation working on training the next young, french speaking, west africans talents in AI and solving real-life problems with their AI skills. In his spare time, Kevin also create programming and AI educational content on Youtube and play video games.
Researcher Profile: Momboladji Balogoun
Momboladji BALOGOUN is the Data Analyst of Gozem, a company providing ride-hailing and other services in West and Central Africa. He is a former Data Scientist at Rintio, an IT startup based in Benin, that uses data and AI to create business solutions for other enterprises. Momboladji holds a M.Sc. degree in Applied Statistics from ICMPA UNESCO Chair, Cotonou, and migrated to the Data Science field after having attended a regional Big Data Bootcamp in his country Benin. He aims to pursue a Ph.D. program on low resources languages speech to speech translation. Bola created Takwimu LAB in August 2019, and he leads it currently with 3 other friends in order to promote Data Science in their countries, but also the creation and the use of AI to solve real-life problems in their communities. His hobbies are: Reading, Documentaries, and Tourism.
Researcher Profile: Godson Kalipe
Godson started in the IT field with software engineering with a specialization on mobile applications. After his bachelor in 2015, he worked for a year as web and mobile application developer before joining a master in India in Big Data Analytics. His master thesis consisted comparative analysis of international news impact on economic indicators of African countries using news Data, Google Cloud storage and visualization assets. After his Master,
in 2019, he gained a first experience as Data Engineer creating data ingestion pipelines for real time sensor data at Activa Inc, India. He parallely has been working with Takwimu Lab on various projects with the aim of bringing AI powered solutions to common african problems and make the field more popular in the west African francophone industry.
Researcher Profile: Jamiil Toure
Jamiil is a design engineer in electrical engineering from Ecole Polytechnique d’Abomey-Calavi (EPAC), Benin in 2015 and a master graduate in mathematical sciences from African School of Mathematical Sciences (AIMS) Senegal in 2018. Passionate of languages and Natural Language Processing (NLP), he contributes to the Masakhane project by working on the creation of a dataset for the language Dendi.
Meanwhile, he complements his education on NLP concepts via online courses, events, conferences for a future research career in NLP. With his friends at Takwimu Lab they work at creating active learning and working environments to foster the applications and usages of AI to tackle real-life problems. Currently, Jamiil is a consultant in Big Data at Cepei – a think tank based in Bogota that promotes dialogue, debate, knowledge and multi-stakeholder participation in global agendas and sustainable development.
Disclaimer
The designations employed and the presentation of material on these map do not imply the expression of any opinion whatsoever on the part of the Secretariat of the United Nations concerning the legal status of any country, territory, city or any area or of its authorities, or concerning the delimitation of its frontiers or boundaries. Final boundary between the Republic of Sudan and the Republic of South Sudan has not yet been determined. Final status of the Abyei area is not yet determined.
Language profile: Fongbe
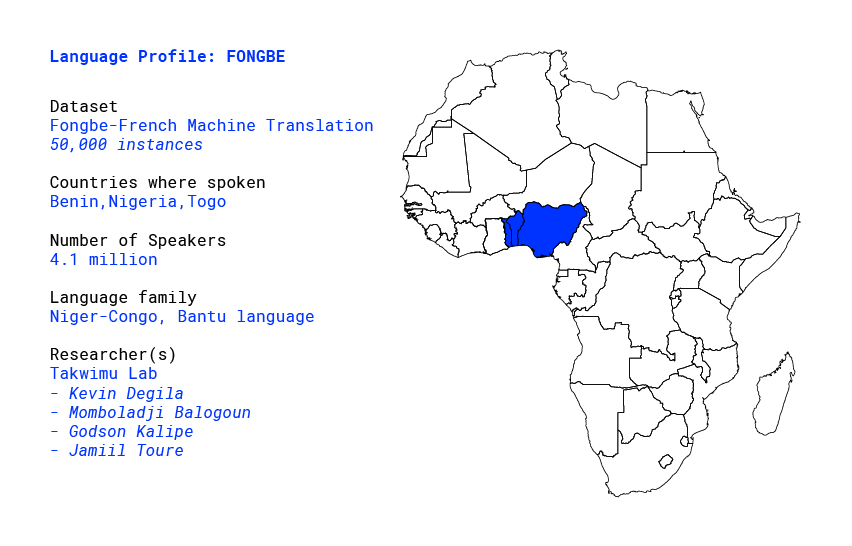
Overview
Fon or fɔ̀ngbè is a low resource language, part of the Eastern Gbe language cluster and
belongs to the Volta–Niger branch of the Niger–Congo languages. Fongbe is spoken in Nigeria, Togo and mainly in Benin by approximately 4.1 million speakers. Like the other Gbe languages, Fongbe is an analytic language with an SVO basic word order. It’s also a tonal language and contains diacritics which makes it difficult to study. [1]
The standardized Fongbe language is part of the Fongbe cluster of languages inside the Eastern Gbe languages. In that cluster, there are other languages like Goun, Maxi, Weme, Kpase which share a lot of vocabulary with the Fongbe language. Standard Fongbe is the primary target of language planning efforts in Benin, although separate efforts exist for Goun, Gen, and other languages of the country. To date, there are about 53 different dialects of the Fon language spoken throughout Benin.
Pertinence
Fongbe holds a special place in the socio economic scene in Benin. It’s the most used language in markets, health care centers, social gatherings, churches, banks, etc.. Most of the ads and some programs on National Television are in Fongbe. French used to be the only language of education in Benin, but in the second decade of the twenty first century, the government is experimenting with teaching some subjects in Benin schools in the country’s local languages, among them Fongbe.
Example of Fongbe Text:
Fongbe : Mǐ kplɔ́n bo xlɛ́ ɖɔ mǐ yí wǎn nú mɛ ɖevo lɛ
English : We have learned to show love to others [3]
Existing Work
Some previous work has been done on the language. There are doctorate thesis, books, French to Fongbe and Fongbe to French dictionaries, blogs and others. Those are sources for written fongbe language.
Researcher Profile: Kevin Degila
Kevin is a Machine Learning Research Engineer at Konta, an AI startup based in Casablanca. he holds an engineering degree in Big Data and AI and it’s currently enrolled in a PhD program focused on business document understanding at Chouaib Doukkali University. In his day to day activities, Kevin train, deploy and monitor in production machine learning models. With his friends, they lead TakwimuLab, an organisation working on training the next young, french speaking, west africans talents in AI and solving real-life problems with their AI skills. In his spare time, Kevin also create programming and AI educational content on Youtube and play video games.
Researcher Profile: Momboladji Balogoun
Momboladji BALOGOUN is the Data Analyst of Gozem, a company providing ride-hailing and other services in West and Central Africa. He is a former Data Scientist at Rintio, an IT startup based in Benin, that uses data and AI to create business solutions for other enterprises. Momboladji holds a M.Sc. degree in Applied Statistics from ICMPA UNESCO Chair, Cotonou, and migrated to the Data Science field after having attended a regional Big Data Bootcamp in his country Benin. He aims to pursue a Ph.D. program on low resources languages speech to speech translation. Bola created Takwimu LAB in August 2019, and he leads it currently with 3 other friends in order to promote Data Science in their countries, but also the creation and the use of AI to solve real-life problems in their communities. His hobbies are: Reading, Documentaries, and Tourism.
Researcher Profile: Godson Kalipe
Godson started in the IT field with software engineering with a specialization on mobile applications. After his bachelor in 2015, he worked for a year as web and mobile application developer before joining a master in India in Big Data Analytics. His master thesis consisted comparative analysis of international news impact on economic indicators of African countries using news Data, Google Cloud storage and visualization assets. After his Master,
in 2019, he gained a first experience as Data Engineer creating data ingestion pipelines for real time sensor data at Activa Inc, India. He parallely has been working with Takwimu Lab on various projects with the aim of bringing AI powered solutions to common african problems and make the field more popular in the west African francophone industry.
Researcher Profile: Jamiil Toure
Jamiil is a design engineer in electrical engineering from Ecole Polytechnique d’Abomey-Calavi (EPAC), Benin in 2015 and a master graduate in mathematical sciences from African School of Mathematical Sciences (AIMS) Senegal in 2018. Passionate of languages and Natural Language Processing (NLP), he contributes to the Masakhane project by working on the creation of a dataset for the language Dendi.
Meanwhile, he complements his education on NLP concepts via online courses, events, conferences for a future research career in NLP. With his friends at Takwimu Lab they work at creating active learning and working environments to foster the applications and usages of AI to tackle real-life problems. Currently, Jamiil is a consultant in Big Data at Cepei – a think tank based in Bogota that promotes dialogue, debate, knowledge and multi-stakeholder participation in global agendas and sustainable development.
Disclaimer
The designations employed and the presentation of material on these map do not imply the expression of any opinion whatsoever on the part of the Secretariat of the United Nations concerning the legal status of any country, territory, city or any area or of its authorities, or concerning the delimitation of its frontiers or boundaries. Final boundary between the Republic of Sudan and the Republic of South Sudan has not yet been determined. Final status of the Abyei area is not yet determined.
Language profile: Yoruba
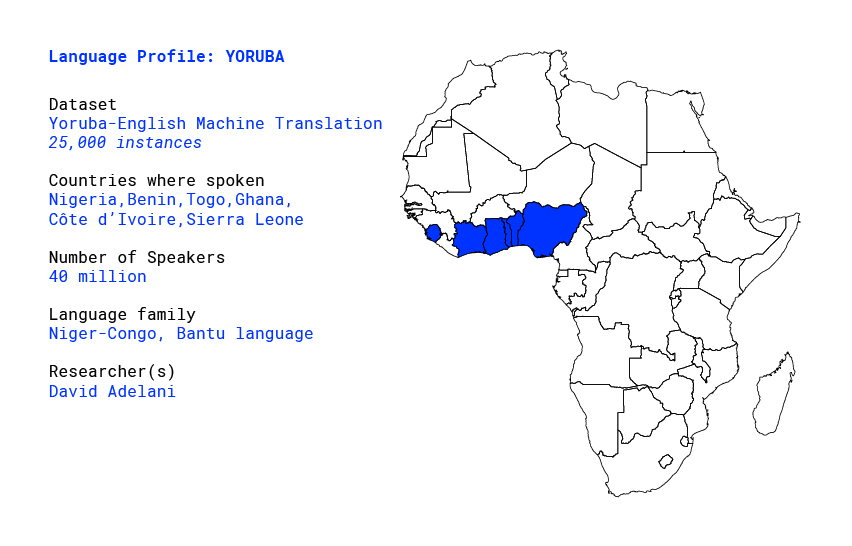
Overview
The Yorùbá language is the third most spoken language in Africa, and is native to the south-western Nigeria and the Republic of Benin in West Africa (as shown in Figure 1). It is one of the national languages in Nigeria, Benin and Togo, and it is also spoken in other countries like Ghana, Côte d’Ivoire, Sierra Leone, Cuba, Brazil and by a significant Yorùbá diaspora population in the US and United Kingdom mostly from the Nigerian ancestry. The language belongs to the Niger-Congo family, and is spoken by over 40 million native speakers [1].
Yorùbá has several dialects but the written language has been standardized by the 1974 Joint Consultative Committee on Education [2], it has 25 letters without the Latin characters (c, q, v, x and z) and with additional characters (ẹ , gb, ṣ , and ọ). There are 18 consonants (b, d, f, g, gb, j, k, l, m, n, p, r, s, s., t, w y), and 7 oral vowels (a, e, ẹ , i, o, ọ , u). Yorùbá is a tonal language with three tones: low, middle and high.
These tones are represented by the grave (“\”), optional macron (“- ”) and acute (“/”) accents respectively. These tones are applied on vowels and syllabic nasals, but the mid tone is usually ignored in writings. The tones are represented in written texts along with a modified Latin alphabet. A few alphabets have underdots (i.e. “ẹ ”, “ọ ”, and “ṣ”), we refer to the tonal marks and underdots as diacritics. It is important to note that tone information is needed for correct pronunciation and to have the meaning of a word [2, 3].
As noted in [4], most of the Yorùbá texts found in websites or public domain repositories either use the correct Yorùbá orthography or replace diacriticized characters with un-diacriticized ones.
Oftentimes, articles written online including news articles1 like BBC and VON ignore diacritics. Ignoring diacritics makes it difficult to identify or pronounce words except they are in a context. For example, owó (money), ọwọ̀ (broom), òwò (business), ọ̀wọ̀ (honour), ọwọ́ (hand), and ọ̀wọ́ (group) will be mapped to owo without diacritics.
Existing work
Due to the problem with the diacritics in Yorùbá language, it has greatly reduced the amount of available parallel texts that can be used for many NLP tasks like machine translation. This has led to research on automatically applying diacritics to Yorùbá texts [5, 6], but the problem has not been completely solved. We will divide the existing work on Yorùbá language into four categories:
Automatic Diacritics Application
The main idea for the automatic diacritic application (ADA) model is to predict the correct diacritics of a word based on the context it appears. We can make use of a sequence-to-sequence deep learning model like Long Short Term Memory networks (LSTM) [7] to achieve this task.
The task is similar to a machine translation task where we need to translate from a source language to a target language, ADA takes a source text that is non-diacriticized (e.g “bi o tile je pe egbeegberun ti pada sile”) and outputs target texts with diacritics (e.g. “bí ó tilẹ̀ jẹ́ pé ẹgbẹẹgbẹ̀rún ti padà síléé”). The first attempt of applying deep learning models to Yorùbá ADA was by Iroro Orife [5].
They proposed a soft-attention seq2seq model to automatically apply diacritics to Yorùbá texts, their model was trained on the Yorùbá bible, Lagos-NWU speech corpus and some language blogs. However, the model does not generalize to other domains like dialog conversation and news domain because the majority of the texts are from the Bible. Orife et al [6] recently addressed the issue of domain-mismatch by gathering texts from various sources like conversation interviews, short stories and proverbs, books, and JW300 Yorùbá texts but they evaluated the performance of the model on the news domain (i.e Global Voices articles) to measure domain generalization.
Word Embeddings
Word embeddings are the primary features used for many downstream NLP tasks. Facebook released FastText [8] word embeddings for over 294 languages 2 but the quality of the embeddings are not very good. Recently, Alabi et. al [9] showed that Facebook’s FastText embeddings for Yorùbá gives a lower performance in word similarity tasks, which indicates that they would not work well for many downstream NLP tasks. They released a better quality FastText embeddings and contextualized BERT [10] embeddings obtained by fine-tuning multi-lingual BERT embeddings.
Datasets for Supervised Learning Tasks
Yorùbá, like many other low-resourced languages, does not have many supervised learning datasets such as named entity recognition (NER), text classification and parallel sentences for machine translation. Alabi et al. [9] created a small NER dataset with 26K tokens. Through the support of AI4D 3 and Zindi Africa 4, we have created parallel English-Yorùbá dataset for machine translation and news title classification dataset for Yorùbá from articles crawled from BBC Yorùbá 5. The summary of the AI4D dataset creation competition is in [11].
Machine Translation
Commercial machine translation models like Google Translate 6 exist for Yorùbá to other languages but the quality is not very good because of the diacritics problem and the small amount of data available to train a good neural machine translation (NMT) model. JW300[12] based on Jehovah Witness publications is another popular dataset for training NMT models for low-resource African languages, it has over 10 million tokens of Yorùbá texts. However, the NMT models trained on JW300, do not generalize to other non-religious domains. There is a need to create more multi-domain parallel datasets for Yorùbá language.
Researcher Profile: David Adelani
David Ifeoluwa Adelani is a doctoral student in computer science at Spoken Language Systems Group, Saarland Informatics Campus, Saarland University, Saarbrücken, Germany. His current research focuses on the security and privacy of users’ information in dialog systems and online social interactions.
He is also actively involved in the development of natural language processing datasets and tools for low-resource languages, with special focus on African languages. He has published a few papers in top Web technology, language and speech conferences including The Web Conference, LREC, and Interspeech.
During his graduate studies, he conducted research on social computing at the Max Planck Institute of Software Systems, Germany and on fake review detection at the National Institute of Informatics, Tokyo, Japan. He holds an MSc in Computer Science from the African University and Science and Technology, Abuja, Nigeria and a BSc in Computer Science from the University of Agriculture, Abeokuta, Nigeria.
Disclaimer
The designations employed and the presentation of material on these map do not imply the expression of any opinion whatsoever on the part of the Secretariat of the United Nations concerning the legal status of any country, territory, city or any area or of its authorities, or concerning the delimitation of its frontiers or boundaries. Final boundary between the Republic of Sudan and the Republic of South Sudan has not yet been determined. Final status of the Abyei area is not yet determined.
Language profil: Chichewa
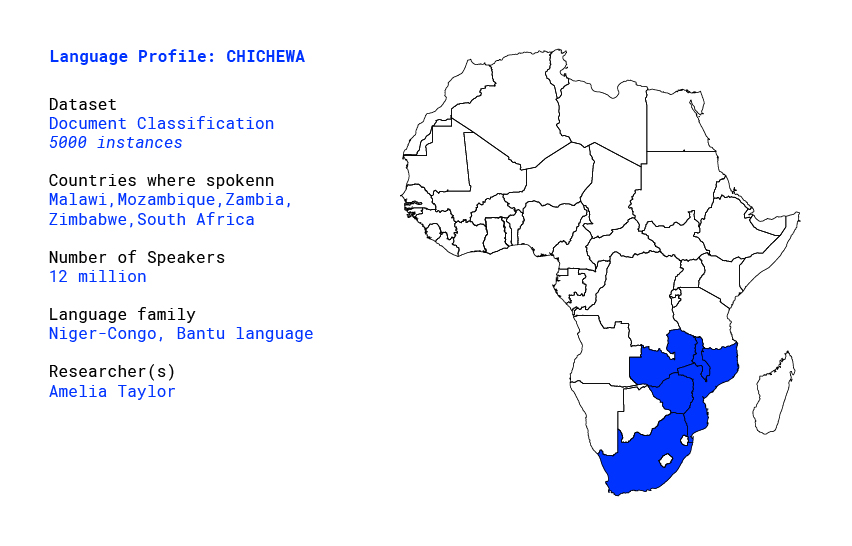
What is Chichewa?
Chichewa is part of the Niger-Congo Bantu group and it is one of the most spoken indigenous languages of Africa. Chichewa is both an individual dialect and a language group as we shall discuss in this short article.
The language, Chichewa, also written as Cichewa, or, in Zambia, Cewa, is the native language of the Chewa. The word ‘chi’ or ‘ci’ is a Bantu prefix used for the tribal name, designating the language rather than the geographical region of the tribe. The word Chewa is the name of a group of people. Chichewa is called Chinyanja, for example in Zambia and Mozambique. Chinyanja was also the old name for the language in Malawi, before the country became a Republic. During that time, as a British Protectorate, Malawi was called Nyasaland.
Chichewa, with the code ‘ny’ is also one of the 13 African languages with a Google automatic translation. The code ‘ny’ was most likely chosen because the language was known first as Chinyanja. This probably reflects the availability of written text in Chichewa compared to other African languages. However, as we will discuss in this article, there are several dialects of Chichewa which differ from each other in noticeable ways. I do not know whether this was taken into account for the text used in the machine language models by Google. But this is a whole new interesting topic in itself!
Who are the Chewa?
The Chewa are a Bantu speaking people, traditionally described as the descendants of the Maravi, who in the 16th (some say, in the 14th) century migrated to the present day Malawi from the region now called Congo-Kinshasa. Most of what we know about the migrations of the Cewa come from oral tradition. Samuel Nthara collected some of the oral traditions in his book Mbiri ya Achewa, published in 1944. The name Maravi first appeared in Portuguese documents in 1661.
Nowadays, some of the well known districts in Malawi where the Chewa live are: Mchinji, Lilongwe, Kasungu, Nkhotakota, Dowa and Dedza. The consensus is that the Chewa of the mainland kept their name as Chewa and lived mainly in the Central Region. The Manganja are the Chewa who settled in the Southern region. And some Chewa groups who settled at the lake or around the Shire River in the south are called Nyanja. Man’ganja (or Maganja) is southern Chichewa as opposed to the language spoken in the Central Region (which was also called Western Chichewa / Nyanja). There are phonetical, grammatical and vocabulary differences between these dialects.
Where is Chichewa spoken?
In Malawi, Chichewa is widely understood. It was declared the national language in 1968 and it is viewed as a symbol of national unity by diverse groups. In Mozambique it is spoken especially in the provinces of Tete and Niassa, where it is referred to as Chinyanja. In Zambia, it is spoken in Lusaka and in the Eastern Province (the language is referred to as Nyanja). The language spoken in Lusaka is sometimes called town-Nyanja as opposed to the Nyanja spoken in rural areas in other parts of Zambia, where it is referred to as deep-Nyanja. Nyanja is the language of the Police and the Army. In Zimbabwe, according to some estimates, Chichewa is the third most widely used language after Shona and Ndebele. There is a sizable community of descendents from those who migrated to this area from Nyasaland during colonial times to work in the mines.
Chichewa is spoken in South Africa. There are a significant number of migrants from Malawi who work in mining, as domestic workers or in other industries. There are radio services in Chichewa in Malawi, Zambia, South Africa and even in Ethiopia.
How many people speak the language?
According to sources quoted in Wikipedia, there are 12 million native speakers of Chichewa. A similar number is mentioned on the Joshua project website and includes Chichewa speakers from 8 countries of the world. This number seems then to refer to all the people who identify themselves as Chewa, Nyanja and Manganja, as these, according to the Malawi Population Census of 2018, make about 40% of the population in Malawi. However, in Malawi, the large ethnic groups of Lomwe, Yao and Ngoni have over the course of time adopted Chichewa as their native language.
It is the case that the number of people understanding and using Chichewa is much higher than the 12 million native speakers. Like Swahili, Chichewa is considered by some a universal language, a common skill enabling people of varying tribes and those living in Malawi, Zambia, Mozambique to communicate without following the strict grammar of specific local languages. In Zambia, many of those whose mother tongue is now Chinyanja have come to consider themselves Ngoni; Nyanja is a lingua franca, being spoken by the police and the administration.
The Need for Datasets in Chichewa
As discussed, seven important facts provide impetus to the initiative to develop data set for Chichewa: (1) Chichewa is an important African language, (2) it is representative of the Niger Congo Bantu group of languages, (3) it is widely spoken, (4) it contains a considerable literature, more than other local African languages, (5) there are several methodological grammar and phonetics studies and (6) several translations from languages such as English and (7) it is spoken by old and young alike.
There has been an interest in developing digital tools for language documentation and natural language processing. Such initiatives have come from researchers involved in linguistics, such as those belonging to linguistics departments at universities in Malawi and Zambia. For example, in Malawi, we found the Chichewa monolingual dictionary corpus containing about 13,000 nouns or this one phonetically annotated short corpus.
The comparative online Bantu dictionary at Berkley includes a dataset for Chichewa, however, the project seems to have stalled in 1997. More recently, there has been an interest in creating datasets used in NLP tools and machine translation and, recently, according to Professor Kishindo, there is a PhD candidate at the University of Malawi interested in working on Machine Translation for Chichewa.
From our investigation, we observe that these datasets or tools tend to be kept in the private domain, are not regularly maintained, or are used only once, and are not well documented. However, their existence is important and it shows that there is a desire and need for such tools.
Conclusions
Chichewa is an important African language. There are differences between the main dialects of Chichewa and the language is undergoing continuous change. Improved methods for discovering online content and digitizing text can open new opportunities for organising Chichewa text into useful corpora. These can then be useful in linguistic work, in building tools for manipulating and comparing text, for finding and visualising connections between texts and for improving machine translation.
Chichewa continues to change as new terms are added to the vocabulary arising from technological needs for example. Its use by the younger generation creates new idioms and meaning, and the creative expressions through poetry and literature find venues online. Looking at language in new and novel ways using technology, can also help engage with the new generation in how they use, view and develop their language.
In this short article, we looked at the use of Chichewa and why we think it is important to build data sets for this language. We hope that this will be motivating and inspiring to others who are interested in this language or other African languages. This article was written as the author embarked on an AI4D Language Dataset Fellowship for putting together a Chichewa dataset. This is a small but important initiative aimed at engaging with the Machine Learning generation on the African continent. I am honoured to be a small part in the building of such datasets.
Researcher Profile: Amelia Taylor
Amelia graduated with a PhD in Mathematical Logic from Heriot-Watt University in 2006 where I was part of the ULTRA group. After that she worked as a research assistant on a project with Heriot-Watt University and the Royal Observatory in Edinburgh, aiming at developing an intelligent query language for astronomical data. From 2006 to 2013, Amelia also worked in finance in the City of London and Edinburgh – she built risk models for asset allocation and liability-driven investments. F
or the last 5 years, Amelia has been teaching programming and AI courses at the University of Malawi in the CIT and engineering department. Amelia also teaches research methodology and supervises MSc and PhD students. While my first interest in AI as an undergraduate was in the field of Natural Language Processing and intelligent query systems, she is interested in the other use of technology and AI for solving real-world problems.
Disclaimer
The designations employed and the presentation of material on these map do not imply the expression of any opinion whatsoever on the part of the Secretariat of the United Nations concerning the legal status of any country, territory, city or any area or of its authorities, or concerning the delimitation of its frontiers or boundaries. Final boundary between the Republic of Sudan and the Republic of South Sudan has not yet been determined. Final status of the Abyei area is not yet determined.
Language profil: Wolof
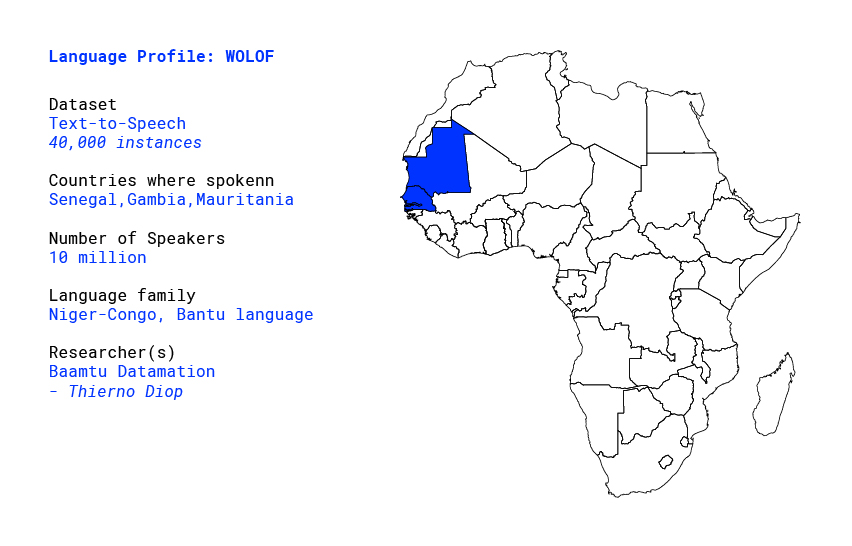
Overview
Wolof /ˈwoʊlɒf/[4] is a language of Senegal, the Gambia and Mauritania, and the native language of the Wolof people. Like the neighbouring languages Serer and Fula, it belongs to the Senegambian branch of the Niger–Congo language family. Unlike most other languages of the Niger-Congo family, Wolof is not a tonal language.[1]
Pertinence
Wolof is spoken by more than 10 million people and about 40 percent (approximately 5 million people) of Senegal’s population speak Wolof as their native language. Increased mobility, and especially the growth of the capital Dakar, created the need for a common language. Today, an additional 40 percent of the population speak Wolof as a second or acquired language. In the whole region from Dakar to Saint-Louis, and also west and southwest of Kaolack, Wolof is spoken by the vast majority of the people. Typically when various ethnic groups in Senegal come together in cities and towns, they speak Wolof. It is therefore spoken in almost every regional and departmental capital in Senegal.[1]
Nevertheless, in Senegal, while the communication in schools and formally registered companies takes place in french, Wolof remains the most used language in critical settings such as :
- Market places
- Medical centers
- In apprenticeship for a major array of occupations such as hairdressing, tailoring, engine
- repairing, carpenting, agriculture among other manual jobs.
- at police stations
- in banking or telecommunications agencies
- in shops and restaurants
Existing work
Senegalease Government has created a linguistic Department for Wolof and other local languages to promote the use of Wolof in some environments like school and also translation of different book in Wolof languages, but there is still a lot of work to have Wolof used in official documents and schools. There also exist some french-wolof dictionaries. In the academic world, some work has been done to better understand Wolof Phonemes[2], POS[3], automatic translation of wolof to french[4], Automatic Speech Recognition From a startup called BAAMTU.
Researcher Profile: Thierno Diop
Thierno Ibrahima DIOP My name is a computer science engineer. He is lead data scientist at Baamtu and passionate about NLP and everything that revolves around machine learning.he has been mentoring data scientist students and apprentices for two years.
Before getting into data science,he did a lot of freelancing in the development of web and mobile applications for local and international clients. he is co-founder of GalsenAI, an artificial intelligence community in Senegal, he is also ZINDI ambassador in Senegal and co-organizer of GDG Dakar.
Disclaimer
The designations employed and the presentation of material on these map do not imply the expression of any opinion whatsoever on the part of the Secretariat of the United Nations concerning the legal status of any country, territory, city or any area or of its authorities, or concerning the delimitation of its frontiers or boundaries. Final boundary between the Republic of Sudan and the Republic of South Sudan has not yet been determined. Final status of the Abyei area is not yet determined.
Language profile: Kiswahili
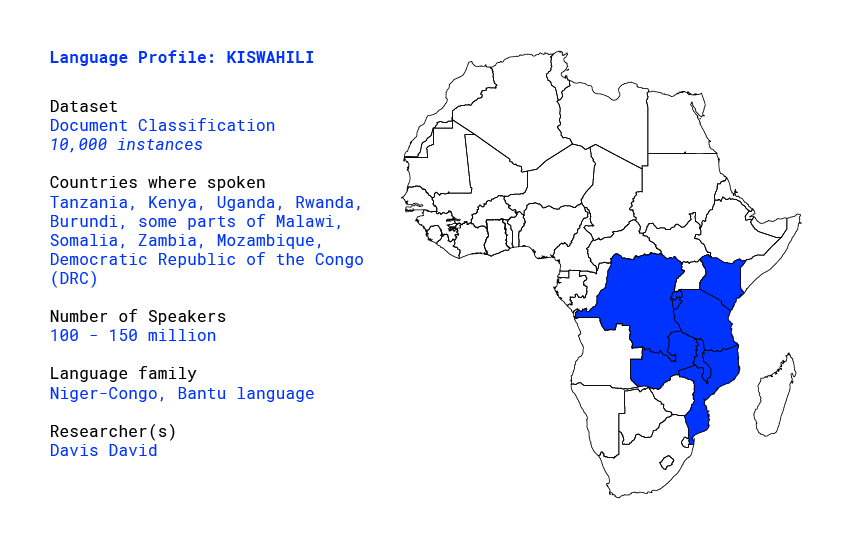
Overview
Swahili (also known as Kiswahili) is one of the most spoken languages in Africa. It is spoken by 100–150 million people across East Africa. Swahili is spoken by countries such as Tanzania, Kenya, Uganda, Rwanda, and Burundi, some parts of Malawi, Somalia, Zambia, Mozambique and the Democratic Republic of the Congo (DRC).
Pertinence
In Tanzania, [1]Swahili is the official language and main communication medium for economic, social, and government activities across the country and it is the official language of instruction in all schools.
Swahili is popularly used as a second language by people across the African continent and taught in schools and universities. Swahili has been influenced by Arabic and even had an Arabic script during its early years., given its presence within the continent and outside.
Swahili is also one of the working languages of the African Union and officially recognized as a lingua franca of the East African Community. In 2018, South Africa legalized the teaching of Swahili in South African schools as an optional subject to begin in 2020. The Southern African Development Community (SADC) officially recognized the Swahili as their official language.
Existing work
In Tanzania, [2]Baraza la Kiswahili la Taifa (National Swahili Council, abbreviated as BAKITA) is a Tanzanian institution responsible for regulating and promoting the Kiswahili language. Key activities mandated for the organization include creating a healthy atmosphere for the development of Kiswahili, encouraging the use of the language in government and business functions, coordinating activities of other organizations involved with Kiswahili, standardizing the language.
BAKITA cooperates with organizations like [3]TATAKI in creation, standardization, and dissemination of specialized terminologies Other institutions can propose new vocabulary to respond to emerging needs but only BAKITA can approve usage. Also, BAKITA coordinates its activities with similar bodies in Kenya and Uganda to aid in the development of Kiswahili.
There exist different English to Swahili dictionaries online from [4]elimuyetu website and Swahili to English dictionaries online from [5]africanlanguages website and the mobile Swahili Dictionary [6] on the Android play store.
Researcher profile: Davis David
He graduated with a Bachelor’s Degree in Computer Science from the University of Dodoma in 2017 where I was a Co-organizer of Python Community during my time at university. After that, he worked as a Software Developer at TYD innovation Incubator developing different innovative systems to solve educational and economical challenges in Tanzania. Davis also worked as a Data scientist at ParrotAI developing different AI solutions focus on Agriculture, health, and finance.
He built computer vision models for classifying Banana Diseases from Leaf Images.. For the last 4 years, Davis has been teaching machine learning and data science across different universities, tech communities, and events with a passion to build a community of Data Scientists in Tanzania to solve local problems
He is also working with Zindi Africa as a Zindi Ambassador and a mentor in Tanzania, he organizes different machine learning hackathons across different cities in Tanzania and mentored different students and junior data scientists across Africa.
Disclaimer
The designations employed and the presentation of material on these map do not imply the expression of any opinion whatsoever on the part of the Secretariat of the United Nations concerning the legal status of any country, territory, city or any area or of its authorities, or concerning the delimitation of its frontiers or boundaries. Final boundary between the Republic of Sudan and the Republic of South Sudan has not yet been determined. Final status of the Abyei area is not yet determined.
Language profile: Tunisian Arabizi
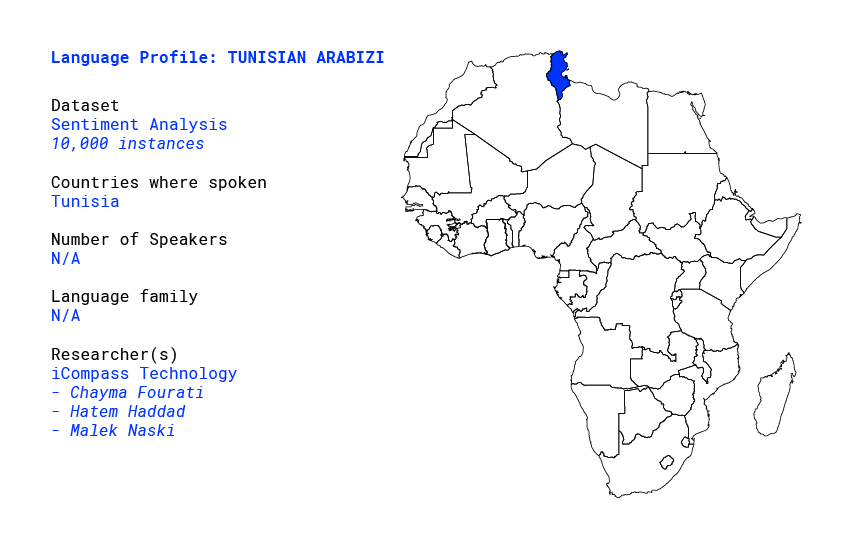
Overview
On Social Media, users tend to express themselves in their own local dialect. To do so, Tunisians use Tunisian Arabizi which consists in supplementing numerals to the Latin script rather than using the Arabic alphabet. [7] mentioned that 81\% of the Tunisian comments on Facebook used the Romanized alphabet.
In [8], a study was conducted on 1,2M social media Tunisian comments (16M words and 1M unique words) showed that 53% of the comments used the Romanized alphabet while 34% used Arabic alphabet and 13% used script-switching.
The study also mentioned that 87% of the comments based on the Romanized alphabet are TUNIZI, while the rest are French and English. TUNIZI, our dataset includes 100% Tunisian Arabizi sentences collected from people expressing themselves in their own local dialect using Latin characters and numerals. TUNIZI is a Sentiment Analysis Tunisian Arabizi Dataset, collected, preprocessed, and annotated
Previous projects on Tunisian Dialect
In [1], a lexicon-based sentiment analysis system was used to classify the sentiment of Tunisian tweets. The author developed a Tunisian morphological analyzer to produce linguistic features and achieved an accuracy of 72.1% using the small-sized TAC dataset (800 Arabic script tweets). [2] presented a supervised sentiment analysis system for Tunisian Arabic script tweets.
With different bag-of-word schemes used as features, binary and multiclass classifications were conducted on a Tunisian Election dataset (TEC)of 3,043 positive/negative tweets combining MSA and Tunisian dialect.
The support vector machine was found of the best results for binary classification with an accuracy of 71.09% and an F-measure of 63%. In [3], the doc2vec algorithm was used to produce document embeddings of Tunisian Arabic and Tunisian Romanized alphabet comments.
The generated embeddings were fed to train a Multi-Layer Perceptron (MLP) classifier where both the achieved accuracy and F-measure values were 78% on the TSAC (Tunisian Sentiment Analysis Corpus) dataset.
This dataset combines 7,366 positive/negative Tunisian Arabic and Tunisian Romanized alphabet Facebook comments. The same dataset was used to evaluate Tunisian code-switching sentiment analysis in [5] using the LSTM-based RNNs model reaching an accuracy of 90%.
In [4], authors conducted a study on the impact on the Tunisian sentiment classification performance when it is combined with other Arabic based pre-processing tasks (Named Entities tagging, stopwords removal, common emoji recognition, etc.).
A lexicon-based approach and the support vector machine model were used to evaluate the performances on the above-mentioned datasets (TEC and TSAC datasets).
In order to avoid the hand-crafted features labor-intensive task, syntax-ignorant n-gram embeddings representation composed and learned using an unordered composition function and a shallow neural model was proposed in [6].The proposed model, called Tw-StAR, was evaluated to predict the sentiment on five Arabic dialect datasets including the TSAC dataset [3].
We observe that none of the existing Tunisian sentiment analysis studies focused on the Tunisian Romanized alphabet which is the aim of this work.
Tunisian Arabizi vs Arabic Arabizi
Tunisian dialect, also known as “Tounsi” or “Derja”, is different from ModernStandard Arabic. In fact, Tunisian dialect features Arabic vocabulary spiced with words and phrases from Tamazight, French, Turkish, Italian and other languages [9].Tunisia is recognized as a high contact culture where online social networks play a key role in facilitating social communication [10].
]. To illustrate more, some examples of Tunisian Arabizi words translated to MSA and English are presented in Table 1.
TUNIZI | MSA translation | English Translation |
3asslema | مرحبا | Hello |
Chna7welek | كيف حالك | How are you |
Sou2el | سؤال | Question |
5dhit | أخذت | I took |
Table 1: Examples of TUNIZI common words translated to MSA and English
Since some Arabic characters do not exist in the Latin alphabet, numerals, and multigraphs instead of diacritics for letters, are used by Tunisians when they write on social media. For instance, ”ch” is used to represent the character ش.
An example is the word شرير (wicked) represented as ”cherrir” in TUNIZI characters. After a few observations from the collected datasets, we noticed that Arabizi used by Tunisians is slightly different from other informal Arabic dialects such as Egyptian Arabizi. This may be due to the linguistic situation specific to each country. In fact, Tunisians generally use the French background when writing in Arabizi, whereas, Egyptians would use English.
For example, the word مشيت would be written as ”misheet” in Egyptian Arabizi, the second language being English. However, because the Tunisian’s second language is French, the same word would be written as ”mchit”.In Table 2, numerals and multigraphs are used to transcribe TUNIZI char-acters that compensate the absence of equivalent Latin characters for exclusively Arabic Arabic sounds.
They are represented with their corresponding Arabic characters and Arabizi characters in other countries. For instance, the number 5 is used to represent the character خ in the same way as the multigraph ”kh”.
For example, the word ”5dhit” is the representation of the word أخذت as shown in Table 1. Numerals and multigraphs used to represent TUNIZI are different from those used to represent Arabizi. As an example, the word غالية (expensive) written as ”ghalia” or ”8alia” in TUNIZI corresponds to ”4’alia” in Arabizi.
Arabic | Arabizi | TUNIZI |
ح | 7 | 7 |
خ | 5 or 7’ | 5 or kh |
ذ | d’ or dh | dh |
ش | $ or sh | ch |
ث | t’ or th or 4 | th |
غ | 4’ | gh or 8 |
ع | 3 | 3 |
ق | 8 | 9 |
Table 2: Special Tunizi characters and their corresponding Arabic and Arabizi characters
Tunizi Uses
TUNIZI dataset can be used for Sentiment Analysis projects dedicated for other underrepresented Maghrebian dialects, such as the Libyan, Moroccan or Algerian because of similarities of the dialects. Also, this dataset can be used also for other NLP projects, such as chatbots.
Tunizi in the industry
TUNIZI dataset is used in all iCompass products that are using the Tunisian Dialect. TUNIZI is used in a Sentiment Analysis project dedicated for the e-reputation and also for all Tunisian chatbots that are able to understand the Tunisian Arabizi and reply using it.
Researcher Profile: Chayma Fourati
Chayma Fourati is an AI R&D Engineer at iCompass. She is a graduate of Software Engineering (June 2020) from the Mediterranean Institute of Technology in Tunisia. She had her final year project at iCompass where she participated in most of the R&D projects. She was invited as a speaker at a webinar during the covid-19 crisis in March 2020 to talk about African IT solutions in fighting the Covid-19 through the latest AI Technologies.
During her last academic years, in both internships and university classes, she developed her skills in the AI field, and at iCompass, in the NLP field. During her final year internship at iCompass, she published a paper with two teammates at iCompass in the ICLR 2020 workshop. Her current research intersts include Natural Language Processing, Neural Networks and Deep Learning.
Researcher Profile: Hatem Haddad
Hatem Haddad is Co-Founder, CTO and RD director of iCompass. He received a doctorate in Computer Science (2002) from University Grenoble Alpes, France. He occupied assistant professor positions at Grenoble Alpes university (France), NTNU (Norway), at UAEU (EAU), at Sousse university (Tunisia), at Mevlana university (Turkey) and at ULB (Belgium). He worked for industrial corporations in R&D at VTT Technical Research Centre of Finland and Institute for Infocomm Research, Image Processing and Applications Lab of Singapore.
He was an invited researcher at Leibniz-Fachhochschule School of Business (Germany) and Polytechnic Institute of Coimbra (Portugal). His current research interests include Natural Languages Processing, Machine Learning and Deep Learning. He is author or co-author of more than 50+ papers published in peer-reviewed international Journals and Conferences and a frequent reviewer for international journals, conferences and R&D projects.
Researcher Profile: Malek Naski
Malek Naski is currently a summer intern at iCompass. She will graduate in June 2021 as a software engineer from the national school of engineering of Tunis (ENIT). Previously, she did her academic end-of-year project for the year 2019/2020 at iCompass, working on sentiment analysis and classification for the tunisian dialect using state-of-the-art NLP methods and technology. She is now focusing on natural language processing and natural language understanding and her current research interests include sentiment analysis and conversational agents.
Disclaimer
The designations employed and the presentation of material on these map do not imply the expression of any opinion whatsoever on the part of the Secretariat of the United Nations concerning the legal status of any country, territory, city or any area or of its authorities, or concerning the delimitation of its frontiers or boundaries. Final boundary between the Republic of Sudan and the Republic of South Sudan has not yet been determined. Final status of the Abyei area is not yet determined.
Language profile: Twi
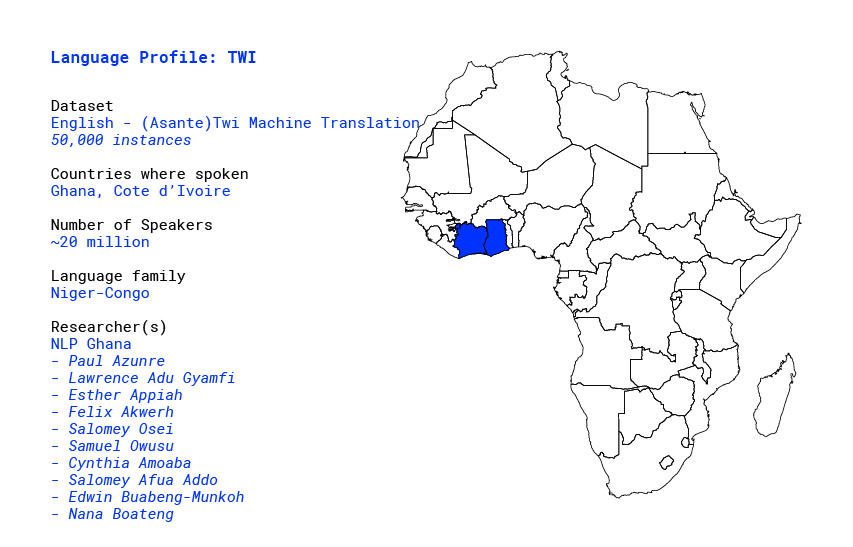
Overview
Twi is arguably the most recognizable Akan Language natively spoken in parts of southern and central Ghana, as well as parts of Cote d’Ivoire. By some estimates it has approximately 20 million native speakers [1]. It is a tonal language. It comprises at least four distinct dialects, namely Asante, Akuapem, Fante and Bono. Asante is arguably the most widely spoken and common dialect.
Pertinence
In practice, knowing this language alone allows one to navigate most parts of Ghana. You are likely to find someone who at the very least understands the language in every part of Ghana.
Example Sentences
English | Twi |
What is going on here? | Ɛdeɛn na ɛrekɔ so wɔ ha? |
Wake up | Sɔre |
She comes here every Friday | Ɔba ha Fiada biara |
Learn to be wise | Sua nyansa |
Prior Work
The website and app Kasahorow [2] has a rather limited set of translations. The JW300 dataset [3] has some (over ½ million) extremely noisy English to (Akuapem) Twi parallel translation sentence pairs. A noisy Wikipedia is available [4], but the volume and quality leave much to be desired [4]. Some 700 sentence pairs are available in the TC Akan Corpus [9].
A recent study [5], which investigated the quality of these data sources in the context of FastText embeddings constructed on Twi, found them to be woefully insufficient. It is the only modern computing study of Twi that we are aware of. We have since replicated and slightly improved these FastText embeddings [6], trained and shared a variety of embeddings from the Transformers/BERT family through the HuggingFace model repo [7] and crowd sourced close to 1000 manually curated translation pairs. We have also developed a fairly decent English-Twi translator (transformer-based seq2seq model) which we are hoping to refine on the data that this collaboration yields. You can find more information on our official and github pages [8].
Researcher Profile: Paul Azunre
Paul Azunre holds a PhD in Computer Science from MIT and has served as a Principal Investigator on several DARPA research programs. He founded Algorine, a Research Lab dedicated to advancing AI/ML and identifying scenarios where they can have a significant social impact. Paul also co-founded NLP Ghana, an open source initiative focused on using NLP and Transfer Learning with Ghanaian and other low-resource languages. He frequently contributes to peer-reviewed journals and has served as a program committee member at some ICML workshops in AutoML and NLP. He is the author of the “Transfer Learning for NLP” book recently published by Manning Publications.
Researcher Profile: Lawrence Adu-Gyamfi
A subsea installation engineer by profession with a background in Aerospace engineering. Currently devoting the rest of my off-work time to contributing to the activities of NLP Ghana, assisting with the collection of data, preprocessing them and making them ready for use in the models we are testing internally. Serving as the NLP Ghana Director of Product, overseeing how the different teams of NLP Ghana work together.
Researcher Profile:Esther Appiah
Esther Appiah holds a BA in Modern Languages from the Kwame Nkrumah University of Science and Technology with a Diploma in French Studies from the Université D’Abomey Calavi, Centre Beninois des Langues Étrangères (CEBELAE) in Benin. She is currently pursuing an MPhil in Theoretical Linguistics at UiT, Norway. Her language specialties include French, English and Akan. She has a vast experience spanning various sectors/industries on language use and interface with core tasks on writing, proofreading, translation and researching. She works with the Ghana NLP as a data researcher and ultimately hopes to specialise in Computational Linguistics to help streamline NLP processes in underrepresented African languages in the digital space.
Researcher Profile: Felix Akwerh
Felix is currently enrolled in a Masters program in Computer Science at the Kwame Nkrumah University of Science and Technology. He augments his education with online classes and Machine Learning events. He is actively involved in the development of natural language processing with Ghana NLP. He co-authored a paper on Artificial Intelligence in Construction for submission. He holds a Bsc in Mathematics at the Kwame Nkrumah University. He worked with the UITS-KNUST where he helped build a transport system and other software projects. His research interest lies in Machine Learning and NLP, specifically in neural conversational models.
Researcher Profile: Salomey Osei
Salomey holds a Master of Philosophy in Applied Mathematics and an Msc in both Industrial Mathematics and Machine Intelligence. She is a recipient of Google and Facebook Scholarship, MasterCard Foundation Scholarship amongst others. She is the team lead for unsupervised methods for Ghana NLP and a co organizer for Women in Machine Learning and Data Science Accra chapter (WiMLDS). She is also passionate about mentoring students, especially females in STEM and her long term goal is to share her knowledge with others by lecturing.
Researcher Profile: Samuel Owusu
Samuel Owusu is currently working as a data scientist for the Ministry of Finance, Ghana. He holds a BSc in Information Technology from Ghana Technology University College. He was a team member of the group that won 1st prize of Ghana’s maiden national hackathon organised by the World Bank and Ministry of Water Resources and Sanitation. His Research interest lies in NLP – Automatic Speech Recognition for low resourced languages. He is involved in developing open source curriculums in Machine Learning and Computer Science for young girls. Samuel is a life-long learner.
Researcher Profile: Cynthia Amoaba
Cynthia Amoaba is a high school graduate from Chemu Senior High School and a student at the University For Development Studies. She’s an Ambassador and founder of the first Women In Stem (WiSTEM) chapter in Ghana.She also founded the STEM club in her high school and looks forward to extending it to schools in deprived areas. Currently, she tutors high school students in her community in Physics and Maths and helps train school dropouts in beads and soap making. She’s a science enthusiast and looks forward to learning more through her involvement in the development of NLP with Ghana-NLP.
Researcher Profile: Salomey Afua Add
Salomey Afua Addo is the founder of Lighted Hope, a Non Governmental Organization that seeks to promote literacy and coding skills among children living in slums in Ghana. She holds an MSc in Mathematical Sciences from the African Institute for Mathematical Sciences and a certificate in business management from the European School of Management and Technology, Berlin. She is the coding instructor for The Love Academy in the USA. Currently, she serves as a volunteer at Ghana NLP, and she plays a vital role in collecting and preprocessing data for the data team at Ghana NLP. Salomey Afua Addo lives a purpose driven life.
Researcher Profile: Edwin Buabeng-Munkoh
Edwin Buabeng-Munkoh is currently working as a Software Engineer at Huawei Technologies Ghana Limited. He holds a BSC in Computer Engineering from Kwame Nkrumah University of Science and Technology. He is enrolled in the Data Science Mentorship program with Notitia AI. He is actively involved in the development of natural language processing with GhanaNLP. He serves as a volunteer at Ghana NLP where he helps with preprocessing data for the data team. Along with his daily work he has enrolled and completed multiple online courses on Data Science, AI and NLP. His research interest lies in Machine Learning, NLP and Computer Vision. He plans to help build a world where language is not a barrier in education and good healthcare
Researcher Profile:Nana Boateng
Nana Boateng holds a PhD. in Statistics from The University of Memphis. He has three masters degrees in Statistics, Mathematics and Economics. He has worked as a Data Scientist for Companies such as Fiat Chrysler Automobiles, Nice Systems Inc and Baptist Memorial Hospital. He is interested in application of mathematics, statistics and economics principles in solving problems in healthcare, finance and several other industries. He has several peer-reviewed publications to his name. He is the founder of Rest Analytics which advises companies on how to apply machine learning to increase efficiency and productivity. He contributes to GhanaNLP in the area of supervised learning.
Partners
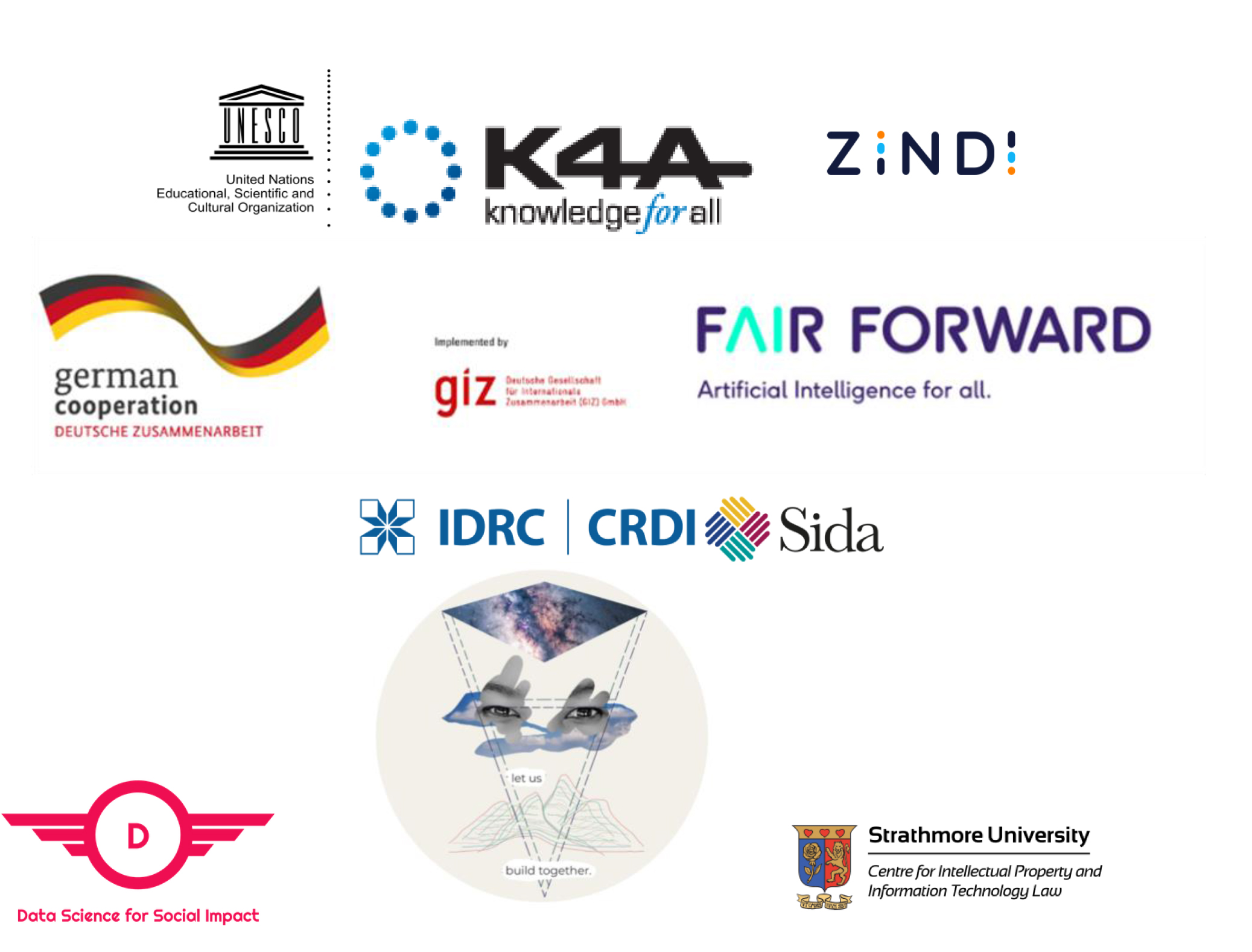
References
[1] https://en.wikipedia.org/wiki/Twi
[2] https://www.kasahorow.org/
[3] Z. Agic et. al., JW300: A Wide-Coverage Parallel Corpus for Low-Resource Languages, ACL Proceedings
[5] J. Alibi et al., Massive vs. Curated Word Embeddings for Low-Resourced Languages. The Case of Yorùbá and Twi, LREC Proceedings 2020
[6] https://medium.com/swlh/ghana-nlp-computational-mapping-of-ghanaian-languages-edf60c56bcce
[7] https://huggingface.co/Ghana-NLP
[8] https://ghananlp.github.io/
[9] https://www.researchgate.net/publication/323998547_TypeCraft_Akan_Corpus_Release_10
Disclaimer
The designations employed and the presentation of material on these map do not imply the expression of any opinion whatsoever on the part of the Secretariat of the United Nations concerning the legal status of any country, territory, city or any area or of its authorities, or concerning the delimitation of its frontiers or boundaries. Final boundary between the Republic of Sudan and the Republic of South Sudan has not yet been determined. Final status of the Abyei area is not yet determined.
Language profile: Luganda
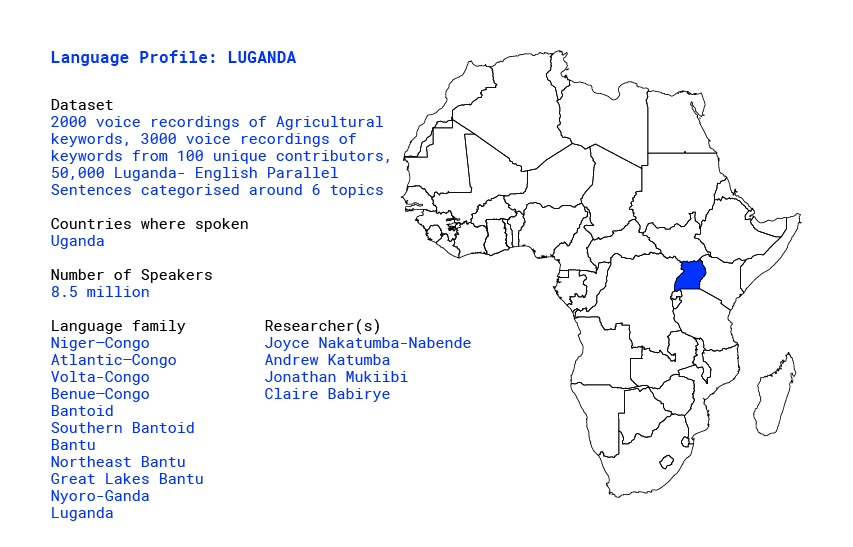
Overview
The Ganda language or Luganda is a Bantu language spoken in the African Great Lakes region. It is one of the major languages in Uganda, spoken by more than eight million Baganda and other people principally in central Uganda, including the capital Kampala of Uganda. It belongs to the Bantu branch of the Niger-Congo language family. Typologically, it is a highly-agglutinating, tonal language with subject-verb-object, word order, and nominative-accusative morphosyntactic alignment [1].
With about six million first-language speakers in the Buganda region and a million others fluent elsewhere, it is the most widely spoken Ugandan language. As a second language, it follows English and precedes Swahili [1].
The language is used in all domains: education, media, telecommunication, trade, entertainment and in religious centres [2]. It has been used at lower institutions as pupils begin to learn English and until the 1960s, Luganda was also the official language of instruction in primary schools in Eastern Uganda [1]. It is also among the languages that have been tabled in the East African parliament to be selected as the official language for the East Africa Community [2].
Existing Work
As the use of the Luganda language is drastically growing across the different sectors from formal to informal, there has been work done on building a Luganda corpus and developing NLP models such as a Luganda text to speech machine [3]; English noun phrase to Luganda translator [4], smart Luganda language translator – given a source text in English it translates it to Luganda automatically [5]. To broaden access to search, a Luganda interface was launched for Google web search [6]. However, some of these applications have been developed based on minimal data.
In terms of language resources, there exists the Luganda Bible [7] which is an online Bible from the Word Project [13] and other religious books from the Jehovah’s Witnesses [12]. There exist some good online Luganda dictionaries like the globe dictionary [10], learn Luganda [9], Luganda phrasebook [9], learn Luganda concise [11] dictionary and Luganda Dictionary [8]. However, most of the available dictionaries are copyrighted and contain just a few word extracts in the language which results in a small representation for a language like Luganda where new words are being created and spoken every year.
Quite recently, a drive has been made by a team of researchers from the Makerere AI Lab [15] to add Luganda to the Common voice platform [14] and it is anticipated that through this project, a large voice dataset for building voice recognition models for Luganda will be generated.
Example of Sentence in Luganda
Luganda: Aboomukyalo bafuna nnyo mu by’obulimi.
English: The people in rural areas benefit a lot from agriculture.
Conclusion
With the regional integration of the East African Community in place, the use of the Luganda language has stretched boundaries from Uganda to the East African community, because most of the native speakers of this language are actively participating in this cooperation. It has been used to support inter-ethnic communication [2]. This, however, stretches beyond East Africa on the other hand.
Therefore, there is a need to build a robust Luganda dataset, which can be made publicly available so that different researchers can use it to build downstream applications such as machine translators, speech recognition machines, chatbots, virtual assistants, sentiment analytic models, in ensuring that information is accessible to all and also addressing some of the local-contextual problems with in the society.
Researcher Profile: Joyce Nakatumba-Nabende
Joyce Nakatumba-Nabende is a lecturer in the Department of Computer Science in Makerere University. She is also the head of the Makerere Artificial Intelligence and Data Science Lab in the College of Computing and Information Sciences. She obtained a PhD in Computer Science from Eindhoven University of Technology, The Netherlands. Her current research interests include Natural Languages Processing, Machine Learning, and Process Mining and Business Process Management. She is co-author of more than 20+ papers published in peer-reviewed international journals and conferences. She has supervised several PhD and Masters students in the field of Computer Science and Information Systems. She is a member of several international AI bodies that include Open for Good Alliance, Feministic AI Network and UN Expert Group Recommendation 3C Group on Artificial Intelligence.
Researcher Profile: Andrew Katumba
Andrew Katumba is a Lecturer in the Department of Electrical and Computer Engineering as well as a senior researcher with netLabs!UG, a research Center of Excellence in Telecommunications and Networking both in the College of Engineering, Design, Art & Technology (CEDAT), Makerere University. Andrew champions the research and applied Artificial Intelligence (AI) activities at netLabs!UG as the lead for the Marconi Society Machine Learning Lab. Andrew holds a PhD in Photonics and Machine Learning from the Gent University, Belgium. He has co-authored 50+ publications in peer-reviewed international journals and conferences and holds 2 patents in neuromorphic computing.
Researcher Profile: Jonathan Mukiibi
Jonathan Mukiibi is a computer science practitioner with a background in software engineering,linguistics, machine learning, big data and natural language processing. Over the past years he has been involved in artificial intelligence based projects like satellite image analysis, radio mining, social media mining, ambulance tracking and traffic which have been successfully implemented to solve real world problems in developing communities. Currently, he is pursuing a Masters in Computer Science at Makerere University where he is also doing research work at the AI and Data Science Research Lab. He is actively working on different NLP tasks but majorly doing research in end-to-end topic classification models for crop pests and disease surveillance from radio recordings.
Researcher Profile: Claire Babirye
Claire Babirye is a computer science professional with vast experience in different computing modules: from computer networks, computer security, network monitoring to machine learning, data science, natural language processing, deep learning technologies and use of technology for improved service delivery. She is a Research Assistant at the AI and Data Science Research Lab Makerere University and her role is to: tap into the revolution to obtain more and better data so as to support development work and humanitarian; support data analytics and visualization to generate patterns on insights on the data; develop machine learning models for classification. Within the domain of NLP, she has worked on tasks that involve: sentiment analysis on social media data and text classification to identify topics of interest from the farmer agricultural data.
References
- https://en.wikipedia.org/wiki/Luganda
- https://www.open.edu/openlearn/languages/more-languages/linguistics/english-squeezing-out-local-languages-uganda
- Nandutu, I., & Mwebaze, E. (2020). Luganda Text-to-Speech Machine. arXiv preprint arXiv:2005.05447.
- https://www.researchgate.net/publication/338036914_Model_for_Translation_of_English_Language_Noun_Phrases_to_Luganda
- https://www.researchgate.net/publication/323682143_Smart_Luganda_Language_Translator
- https://africa.googleblog.com/2009/07/how-volunteer-translators-impact-local.html
- https://play.google.com/store/apps/details?id=com.LugandaBible&hl=en
- https://web.archive.org/web/20080120211744/http://www.cbold.ddl.ish-lyon.cnrs.fr/CBOLD_Lexicons/Ganda.Snoxall1967/Text/Ganda.Snoxall1967.txt
- https://learn-luganda.com/
- https://glosbe.com/lg/en/
- https://learnluganda.com/concise
- https://wol.jw.org/lg/wol/h/r138/lp-lu
- https://www.wordproject.org/bibles/lug/index.htm
- https://commonvoice.mozilla.org/lg
- http://www.air.ug/natural-language-processing/