Description
This dataset is part of a 3-4 month Fellowship Program within the AI4D – African Language Program, which was conceptualized as part of a roadmap to work towards better integration of African languages on digital platforms, in aid of lowering the barrier of entry for African participation in the digital economy.
This particular dataset is being developed through a process covering a variety of languages and NLP tasks, in particular a Sentiment Analysis datase of Arabizi.
Language profile: Tunisian Arabizi
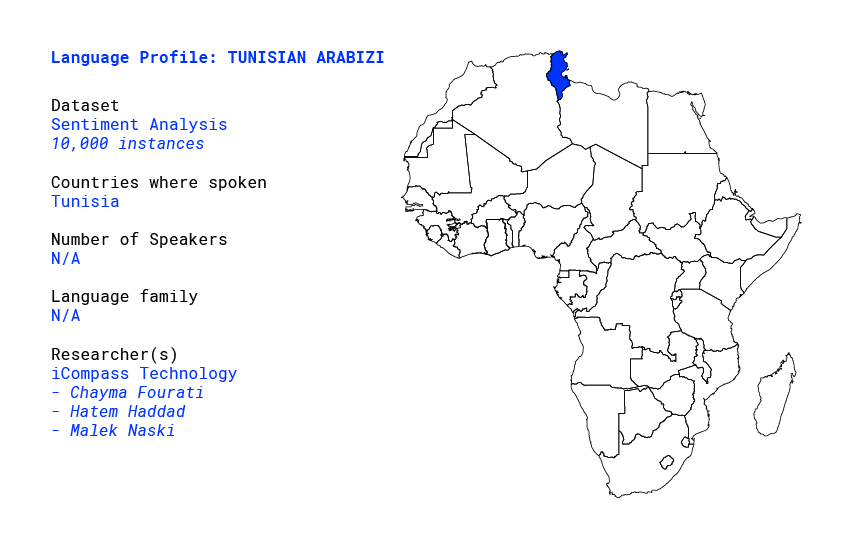
Overview
On Social Media, users tend to express themselves in their own local dialect. To do so, Tunisians use Tunisian Arabizi which consists in supplementing numerals to the Latin script rather than using the Arabic alphabet. [7] mentioned that 81\% of the Tunisian comments on Facebook used the Romanized alphabet.
In [8], a study was conducted on 1,2M social media Tunisian comments (16M words and 1M unique words) showed that 53% of the comments used the Romanized alphabet while 34% used Arabic alphabet and 13% used script-switching.
The study also mentioned that 87% of the comments based on the Romanized alphabet are TUNIZI, while the rest are French and English. TUNIZI, our dataset includes 100% Tunisian Arabizi sentences collected from people expressing themselves in their own local dialect using Latin characters and numerals. TUNIZI is a Sentiment Analysis Tunisian Arabizi Dataset, collected, preprocessed, and annotated
Previous projects on Tunisian Dialect
In [1], a lexicon-based sentiment analysis system was used to classify the sentiment of Tunisian tweets. The author developed a Tunisian morphological analyzer to produce linguistic features and achieved an accuracy of 72.1% using the small-sized TAC dataset (800 Arabic script tweets). [2] presented a supervised sentiment analysis system for Tunisian Arabic script tweets.
With different bag-of-word schemes used as features, binary and multiclass classifications were conducted on a Tunisian Election dataset (TEC)of 3,043 positive/negative tweets combining MSA and Tunisian dialect.
The support vector machine was found of the best results for binary classification with an accuracy of 71.09% and an F-measure of 63%. In [3], the doc2vec algorithm was used to produce document embeddings of Tunisian Arabic and Tunisian Romanized alphabet comments.
The generated embeddings were fed to train a Multi-Layer Perceptron (MLP) classifier where both the achieved accuracy and F-measure values were 78% on the TSAC (Tunisian Sentiment Analysis Corpus) dataset.
This dataset combines 7,366 positive/negative Tunisian Arabic and Tunisian Romanized alphabet Facebook comments. The same dataset was used to evaluate Tunisian code-switching sentiment analysis in [5] using the LSTM-based RNNs model reaching an accuracy of 90%.
In [4], authors conducted a study on the impact on the Tunisian sentiment classification performance when it is combined with other Arabic based pre-processing tasks (Named Entities tagging, stopwords removal, common emoji recognition, etc.).
A lexicon-based approach and the support vector machine model were used to evaluate the performances on the above-mentioned datasets (TEC and TSAC datasets).
In order to avoid the hand-crafted features labor-intensive task, syntax-ignorant n-gram embeddings representation composed and learned using an unordered composition function and a shallow neural model was proposed in [6].The proposed model, called Tw-StAR, was evaluated to predict the sentiment on five Arabic dialect datasets including the TSAC dataset [3].
We observe that none of the existing Tunisian sentiment analysis studies focused on the Tunisian Romanized alphabet which is the aim of this work.
Tunisian Arabizi vs Arabic Arabizi
Tunisian dialect, also known as “Tounsi” or “Derja”, is different from ModernStandard Arabic. In fact, Tunisian dialect features Arabic vocabulary spiced with words and phrases from Tamazight, French, Turkish, Italian and other languages [9].Tunisia is recognized as a high contact culture where online social networks play a key role in facilitating social communication [10].
]. To illustrate more, some examples of Tunisian Arabizi words translated to MSA and English are presented in Table 1.
TUNIZI | MSA translation | English Translation |
3asslema | مرحبا | Hello |
Chna7welek | كيف حالك | How are you |
Sou2el | سؤال | Question |
5dhit | أخذت | I took |
Table 1: Examples of TUNIZI common words translated to MSA and English
Since some Arabic characters do not exist in the Latin alphabet, numerals, and multigraphs instead of diacritics for letters, are used by Tunisians when they write on social media. For instance, ”ch” is used to represent the character ش.
An example is the word شرير (wicked) represented as ”cherrir” in TUNIZI characters. After a few observations from the collected datasets, we noticed that Arabizi used by Tunisians is slightly different from other informal Arabic dialects such as Egyptian Arabizi. This may be due to the linguistic situation specific to each country. In fact, Tunisians generally use the French background when writing in Arabizi, whereas, Egyptians would use English.
For example, the word مشيت would be written as ”misheet” in Egyptian Arabizi, the second language being English. However, because the Tunisian’s second language is French, the same word would be written as ”mchit”.In Table 2, numerals and multigraphs are used to transcribe TUNIZI char-acters that compensate the absence of equivalent Latin characters for exclusively Arabic Arabic sounds.
They are represented with their corresponding Arabic characters and Arabizi characters in other countries. For instance, the number 5 is used to represent the character خ in the same way as the multigraph ”kh”.
For example, the word ”5dhit” is the representation of the word أخذت as shown in Table 1. Numerals and multigraphs used to represent TUNIZI are different from those used to represent Arabizi. As an example, the word غالية (expensive) written as ”ghalia” or ”8alia” in TUNIZI corresponds to ”4’alia” in Arabizi.
Arabic | Arabizi | TUNIZI |
ح | 7 | 7 |
خ | 5 or 7’ | 5 or kh |
ذ | d’ or dh | dh |
ش | $ or sh | ch |
ث | t’ or th or 4 | th |
غ | 4’ | gh or 8 |
ع | 3 | 3 |
ق | 8 | 9 |
Table 2: Special Tunizi characters and their corresponding Arabic and Arabizi characters
Tunizi Uses
TUNIZI dataset can be used for Sentiment Analysis projects dedicated for other underrepresented Maghrebian dialects, such as the Libyan, Moroccan or Algerian because of similarities of the dialects. Also, this dataset can be used also for other NLP projects, such as chatbots.
Tunizi in the industry
TUNIZI dataset is used in all iCompass products that are using the Tunisian Dialect. TUNIZI is used in a Sentiment Analysis project dedicated for the e-reputation and also for all Tunisian chatbots that are able to understand the Tunisian Arabizi and reply using it.
Researcher Profile: Chayma Fourati
Chayma Fourati is an AI R&D Engineer at iCompass. She is a graduate of Software Engineering (June 2020) from the Mediterranean Institute of Technology in Tunisia. She had her final year project at iCompass where she participated in most of the R&D projects. She was invited as a speaker at a webinar during the covid-19 crisis in March 2020 to talk about African IT solutions in fighting the Covid-19 through the latest AI Technologies.
During her last academic years, in both internships and university classes, she developed her skills in the AI field, and at iCompass, in the NLP field. During her final year internship at iCompass, she published a paper with two teammates at iCompass in the ICLR 2020 workshop. Her current research intersts include Natural Language Processing, Neural Networks and Deep Learning.
Researcher Profile: Hatem Haddad
Hatem Haddad is Co-Founder, CTO and RD director of iCompass. He received a doctorate in Computer Science (2002) from University Grenoble Alpes, France. He occupied assistant professor positions at Grenoble Alpes university (France), NTNU (Norway), at UAEU (EAU), at Sousse university (Tunisia), at Mevlana university (Turkey) and at ULB (Belgium). He worked for industrial corporations in R&D at VTT Technical Research Centre of Finland and Institute for Infocomm Research, Image Processing and Applications Lab of Singapore.
He was an invited researcher at Leibniz-Fachhochschule School of Business (Germany) and Polytechnic Institute of Coimbra (Portugal). His current research interests include Natural Languages Processing, Machine Learning and Deep Learning. He is author or co-author of more than 50+ papers published in peer-reviewed international Journals and Conferences and a frequent reviewer for international journals, conferences and R&D projects.
Researcher Profile: Malek Naski
Malek Naski is currently a summer intern at iCompass. She will graduate in June 2021 as a software engineer from the national school of engineering of Tunis (ENIT). Previously, she did her academic end-of-year project for the year 2019/2020 at iCompass, working on sentiment analysis and classification for the tunisian dialect using state-of-the-art NLP methods and technology. She is now focusing on natural language processing and natural language understanding and her current research interests include sentiment analysis and conversational agents.
Partners
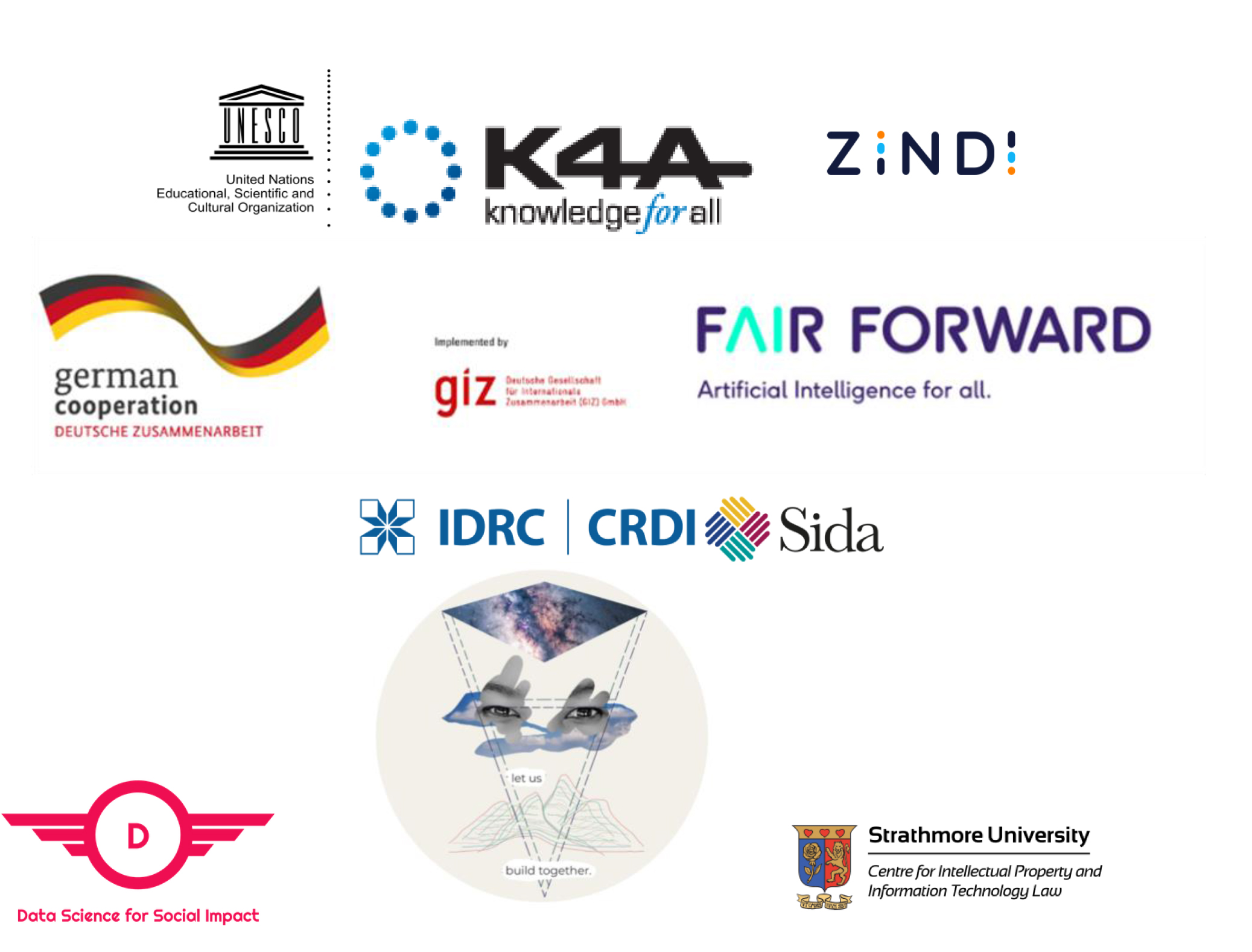
Disclaimer
The designations employed and the presentation of material on these map do not imply the expression of any opinion whatsoever on the part of the Secretariat of the United Nations concerning the legal status of any country, territory, city or any area or of its authorities, or concerning the delimitation of its frontiers or boundaries. Final boundary between the Republic of Sudan and the Republic of South Sudan has not yet been determined. Final status of the Abyei area is not yet determined.